This article was written by Liz Lohn, and originally published in the Medium blog.
Every wave of new technology brings two risks: missing opportunities or chasing hype. Amid the AI frenzy at the start of last year, we were determined to avoid falling into the trap of pursuing a ‘solution looking for a problem.’ Instead, we set out to address meaningful challenges that advances in AI had made possible.
It’s an ambitious yet straightforward mission. But when the scope is so broad, the first challenge becomes clear: where do you start?
Collaboration: The key to clarity
To define our focus, we brought together a cross-functional working group, including colleagues from the newsroom, product, research, engineering, and data science. At these workshops, we achieved three things:
- Shared understanding: We levelled the informational playing field, building a shared view of AI’s potential and its limitations. This helped bridge the gap between those hesitant to engage with AI and those fearing they were missing out on cutting-edge developments.
- Core principles: We established key principles to guide our approach, such as never risking readers’ trust by deploying AI-generated content without rigorous testing. Experimentation, we agreed, would remain contained, closely monitored, and adaptable — ready to pivot or pause as needed.
- A focused remit: By aligning on definitions and terms, we sped up future discussions and agreed on an initial focus: internal newsroom tools to support two stages of the content lifecycle — uncovering and developing stories (more on that in a separate post), and presenting them to readers.
These foundations set the stage for our first experiments.
Starting Small: A Case Study
At one of the workshops, we brainstormed dozens of ideas in the space of story presentation. To prioritise, we asked a key question: Are current AI outputs good enough to produce real value for the newsroom? That value might take the form of efficiency gains or other benefits.
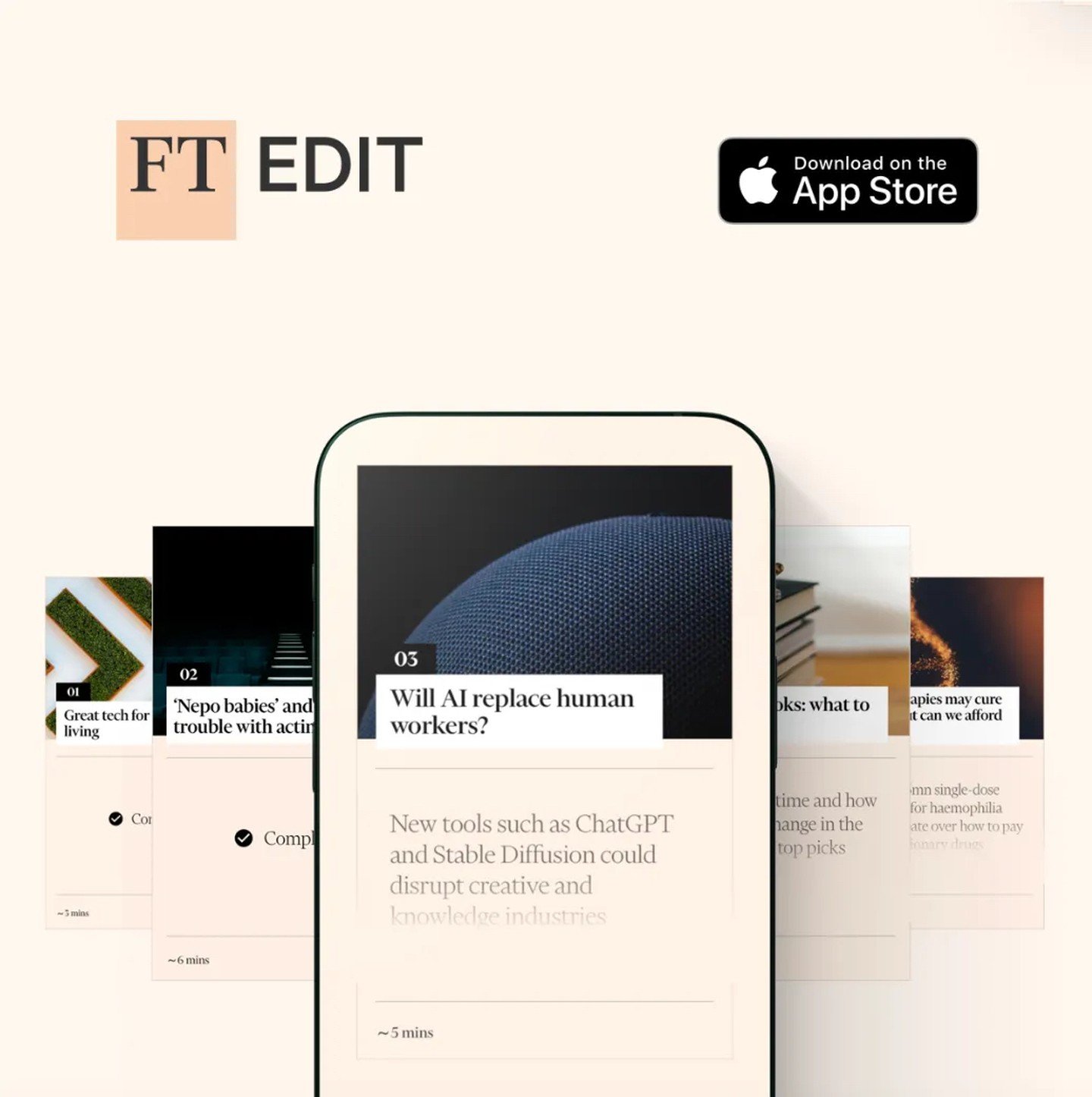
However, we quickly realised we lacked sufficient experience — within the FT or across the industry — to answer this confidently. So, we chose a small, focused use case to explore further: generating summaries for a single newsletter, targeting one specific type of article.
The main challenge? Translating an editor’s judgment into a prompt an LLM could understand. This meant defining how editors identify the most important points of an article, balancing informativeness with engagement, and maintaining both the editor’s voice and the FT’s broader style.
Refining prompts, testing various LLMs, and iterating based on feedback produced a functioning, but ultimately underwhelming, result. The time it took editors to review AI-generated drafts was comparable to writing them from scratch. On top of that, the process of reaching this point was slow — over six weeks — while the AI landscape continued to evolve rapidly.
This raised a critical question: were we really “accelerating” AI experimentation, as the team name promised?
Pivoting to the AI Playground
Realising we needed a faster, more flexible approach, we pivoted. The result was the AI Playground — an internal tool designed to empower the newsroom and other teams to experiment with their own use cases.
Here’s how it works:
- Search and Select: Users can search for FT content using natural language or manually select specific articles.
- Prompt Building: Users can build prompts from scratch or use templates.
- Generate and Rate: The system generates outputs, which users can evaluate for quality and relevance.
- Share and Save: Successful prompts can be saved and shared with colleagues.
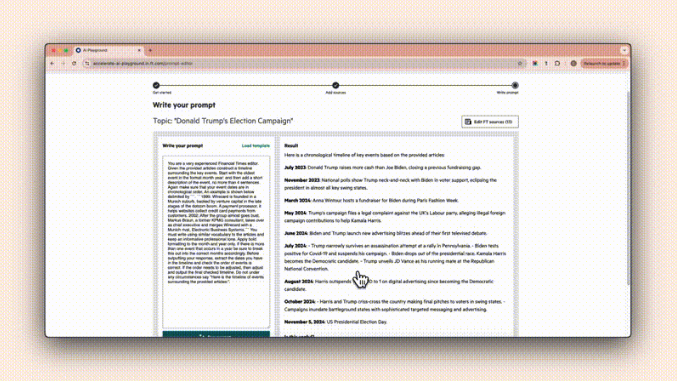
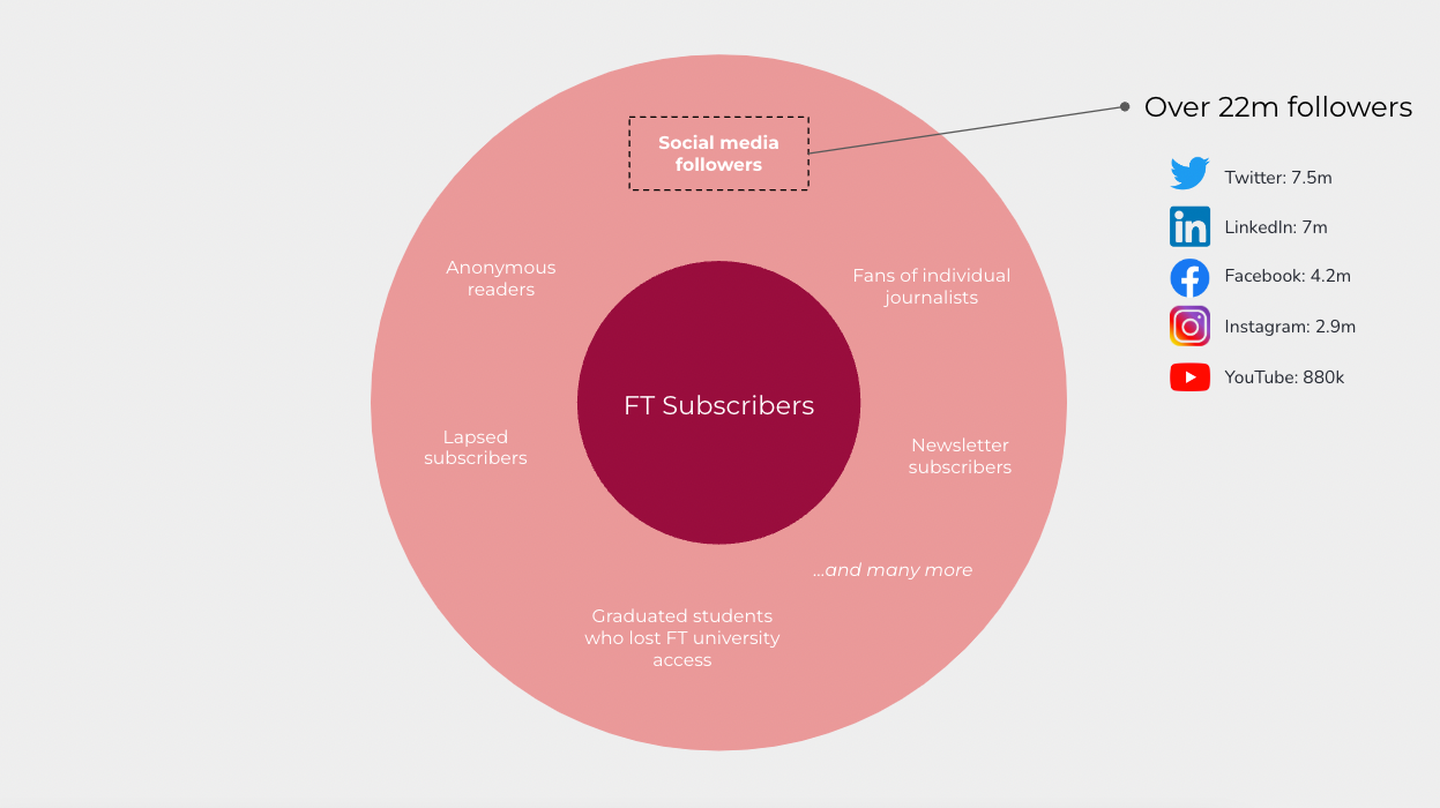
To support experimentation, we embedded best practices into the tool’s system prompts and provided training to help users craft effective queries.
What We’ve Learned
By enabling multiple newsroom users to experiment with various use cases simultaneously, the AI Playground has significantly accelerated our learning. Some of the key insights include:
- Hallucinations are a feature, not a bug: LLMs generate outputs based on statistical probabilities, meaning they can produce convincing but inaccurate information. For a newsroom committed to accuracy, this remains a major hurdle.
- Single-article context works best: While we were initially drawn to use cases requiring multiple articles as input — such as obituaries, timelines, or explainers — we found that outputs often failed to meet quality standards. Conflicting or loosely connected information from multiple sources tended to confuse generative AI. By contrast, single-article inputs produced far better results.
- Viable use cases are limited but valuable: The most promising applications fall into three categories:
- Quality and accuracy gains: Tasks like spotting errors, identifying potential bias, or flagging facts that require checking.
- Low-risk outputs: Generating machine-facing content, such as SEO metadata.
- Inspiration: Helping overcome the “blank page” problem, such as generating conversation starters for online communities.
The Road Ahead
The AI Playground has been transformative, enabling faster, more extensive experimentation and directly involving newsroom experts in the development process. However, the most important lesson we’ve learned is that ‘accelerating AI’ isn’t just about speed — it’s about fostering the right conditions for thoughtful and meaningful progress.
While effective for experimentation, the AI Playground sits outside habitual editorial workflows. Switching between tools diminishes the perceived value of solutions and, ultimately, hinders engagement. Addressing this to encourage sustained use in the newsroom is one of our key challenges.
Looking ahead to 2025, we are also exploring ways AI could empower readers — giving them tools to summarise, simplify, or translate FT content as they choose. Challenges remain, but we’re optimistic that careful experimentation will continue to unlock meaningful opportunities for our journalism and our audience.
At FT Strategies, we have a deep knowledge of AI, technology & data, and what you need to future-proof your business. If you would like to learn more about our expertise and how we can help your company grow, please get in touch.