Personalisation has become the default for digital experiences. Seventy-one percent of consumers now expect companies to deliver personalised interactions. Most businesses have attempted to meet this expectation by investing in this capability, but there are signs that expectations are not being met. A study by Twilio Segment found that although 85% of businesses studied believe they are offering personalised experiences, only 60% of consumers agree.
The technology that makes personalisation possible is constantly evolving. At FT Strategies, we believe that all businesses should invest in continuous improvement of this capability. In this article, we explore the technological maturity curve of personalisation. However mature your technological capabilities might be, we believe there is value in all of the tactics discussed below.
1. Starting out: Active personalisation
Active personalisation is a relatively straightforward way to give customers a more engaging experience.
Active personalisation is when users provide input that results in a personalised experience. For example, an app or service might ask you to specify or share your location, in return for receiving content that’s tailored to that information (e.g. local weather rather than a generic national forecast).
In the publishing world, one of the most successful examples of active personalisation is MyFT. This Financial Times product feature allows subscribers to follow topics of interest. Based on this input, readers can access a customised area on the FT website and apps. They also receive daily email digests based on their stated preferences.
Even this relatively straightforward application of personalisation can have a huge business impact. MyFT remains the FT’s biggest engagement driver. The feature led to an average 86% increase in an individual’s RFV score compared with a control group (for more information about RFV, read our blog post How the FT went from no digital presence to over a million paid digital subscribers’). Similar success has been found at other publishers too. Active personalisation led to engagement increasing by 60% at Gannett and 23% at Mediahuis.
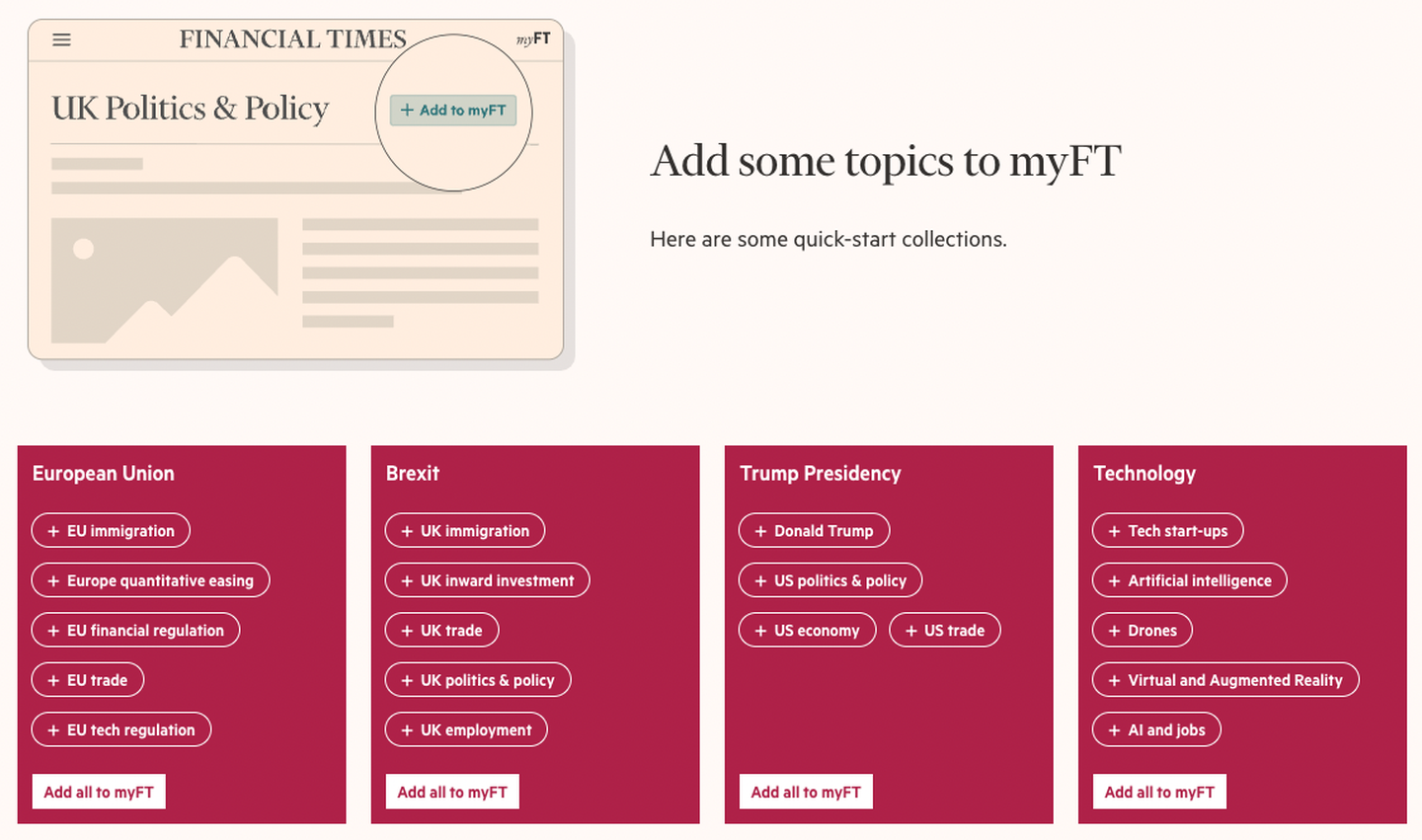
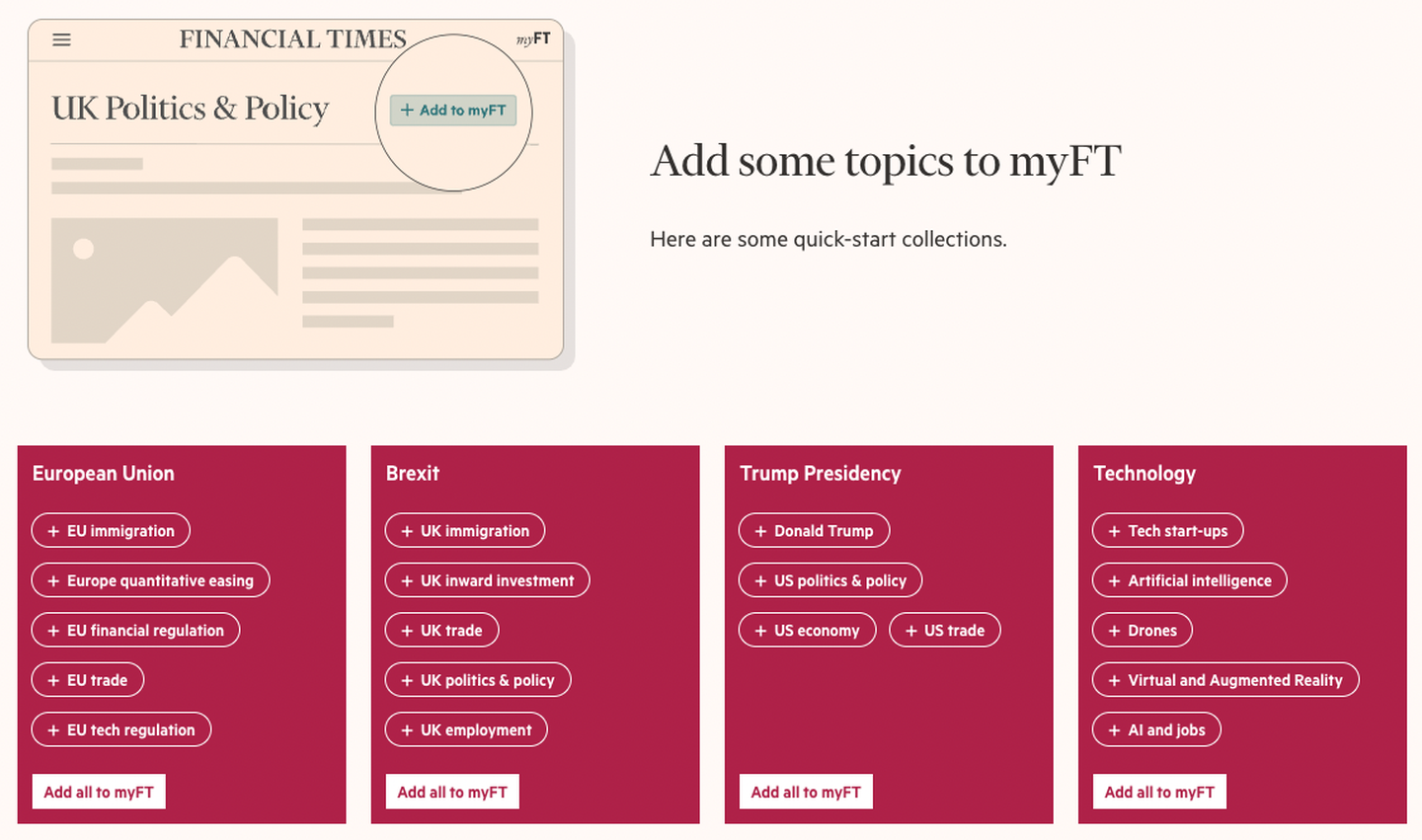
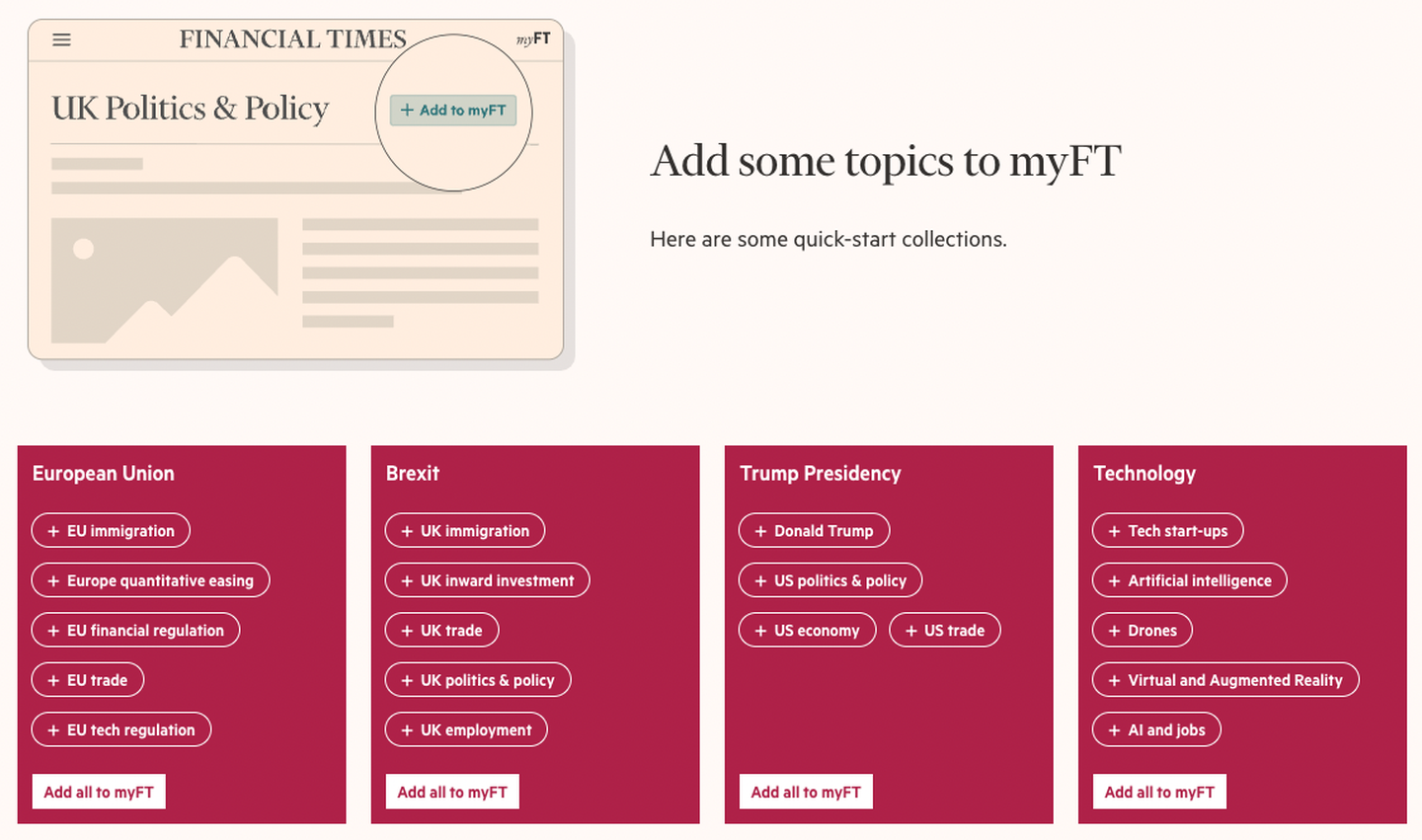
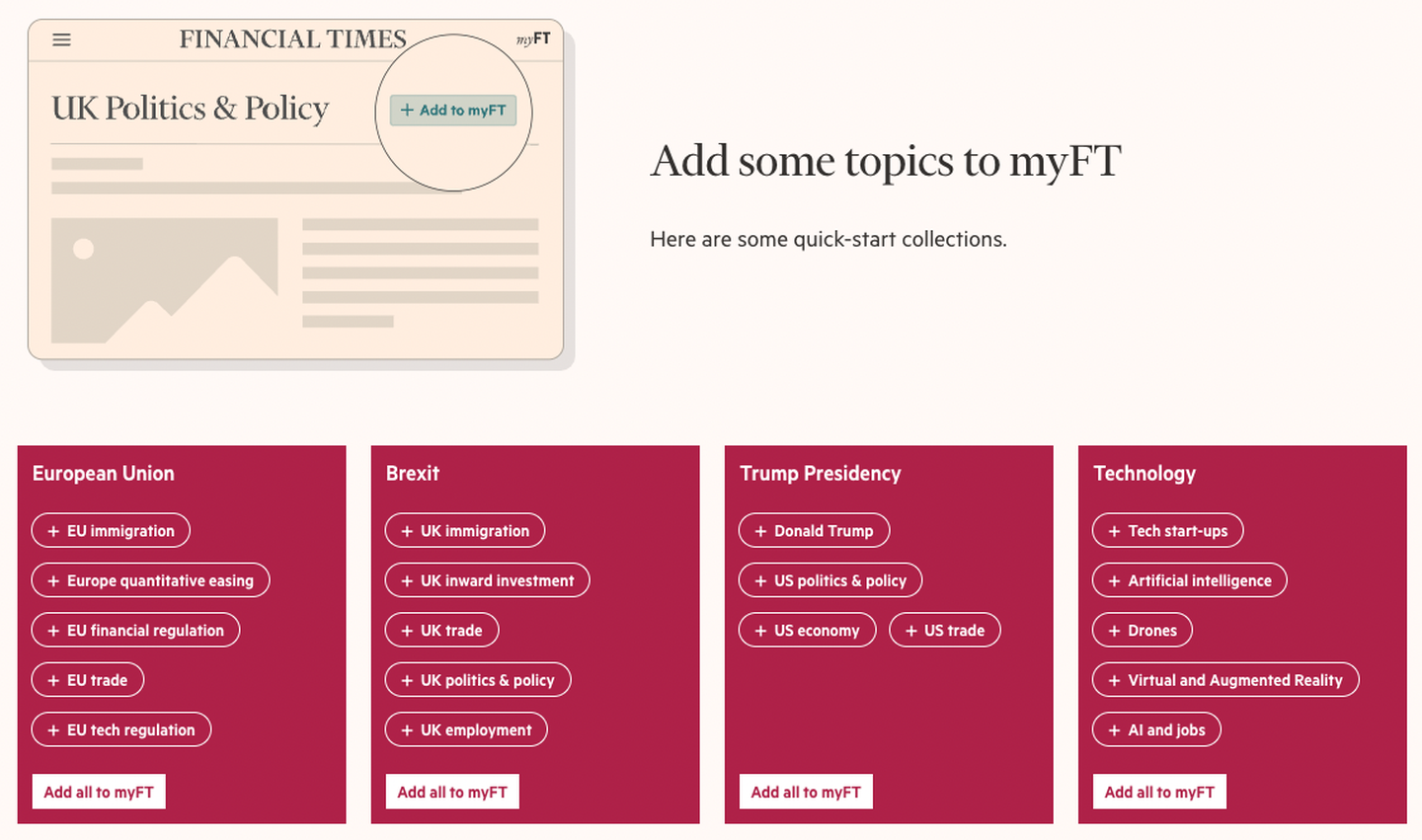
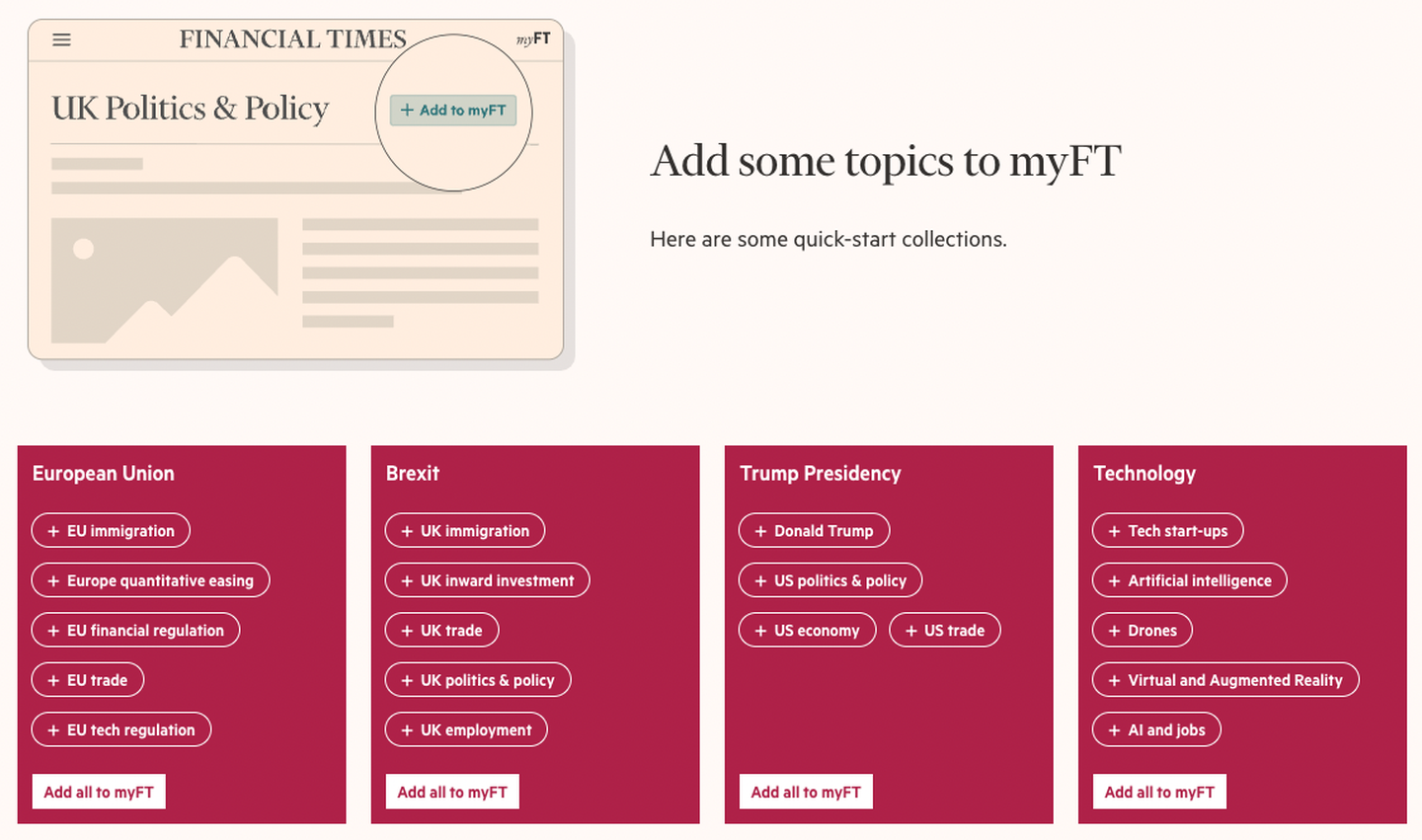
Active personalisation also provides a useful complement to more sophisticated types of personalisation. Increasingly, this approach is used during onboarding flows. For example, when you sign up for Spotify you are asked to choose from a list of artists. This input allows Spotify to immediately provide tailored playlists and recommendations. Over time, Spotify collects listening data to power more sophisticated ‘passive personalisation’, hopefully leading to more accurate recommendations.
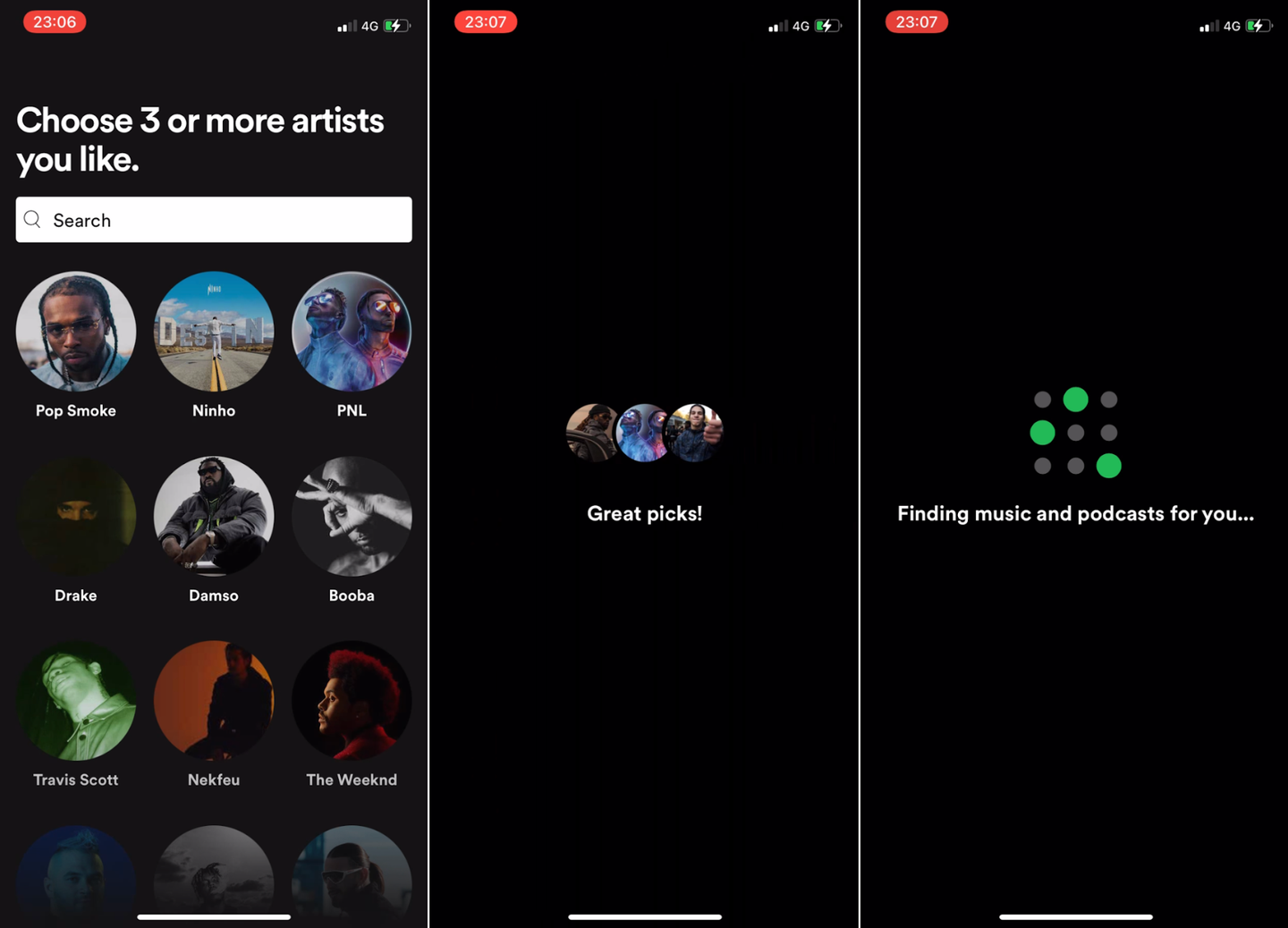
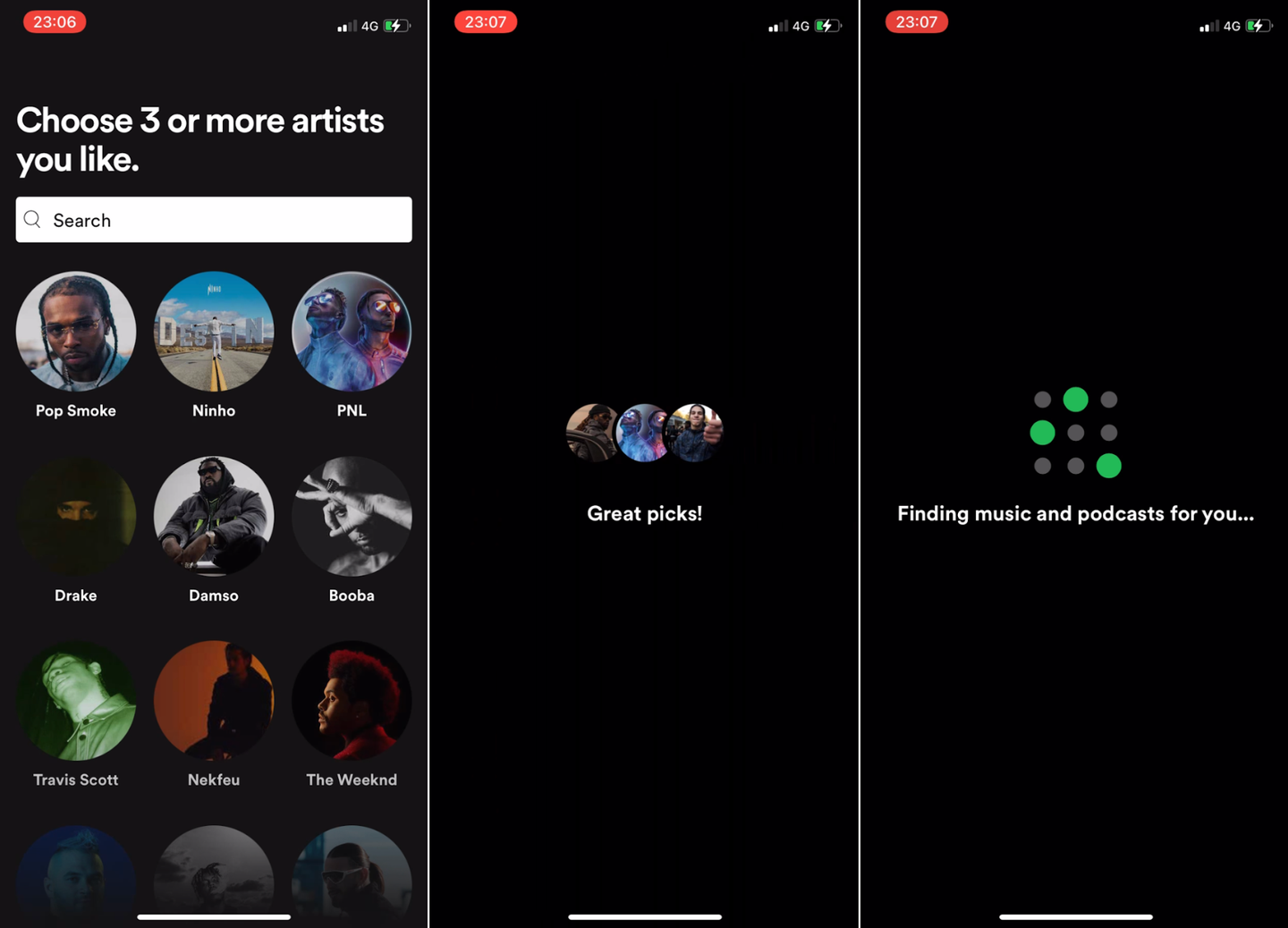
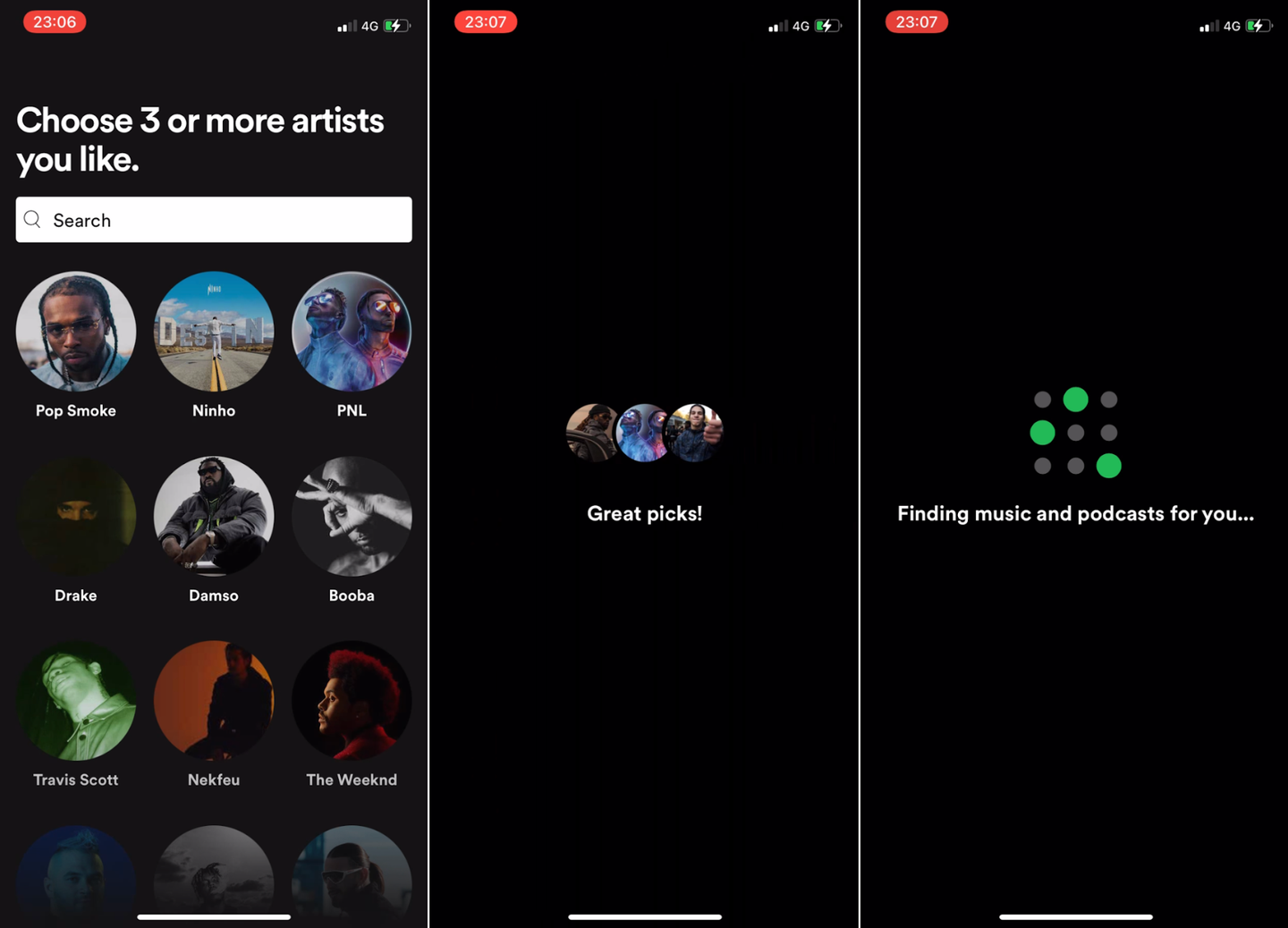
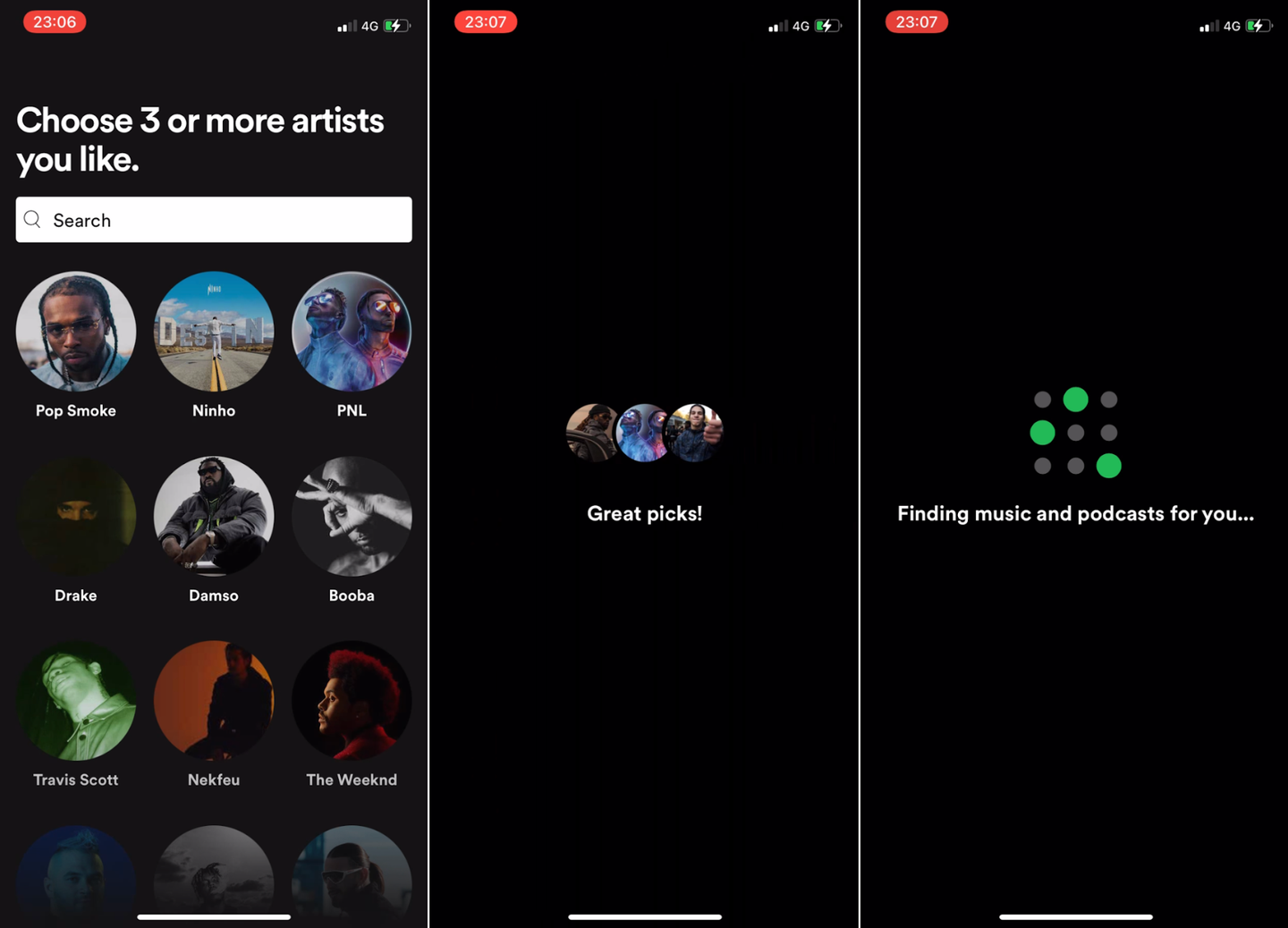
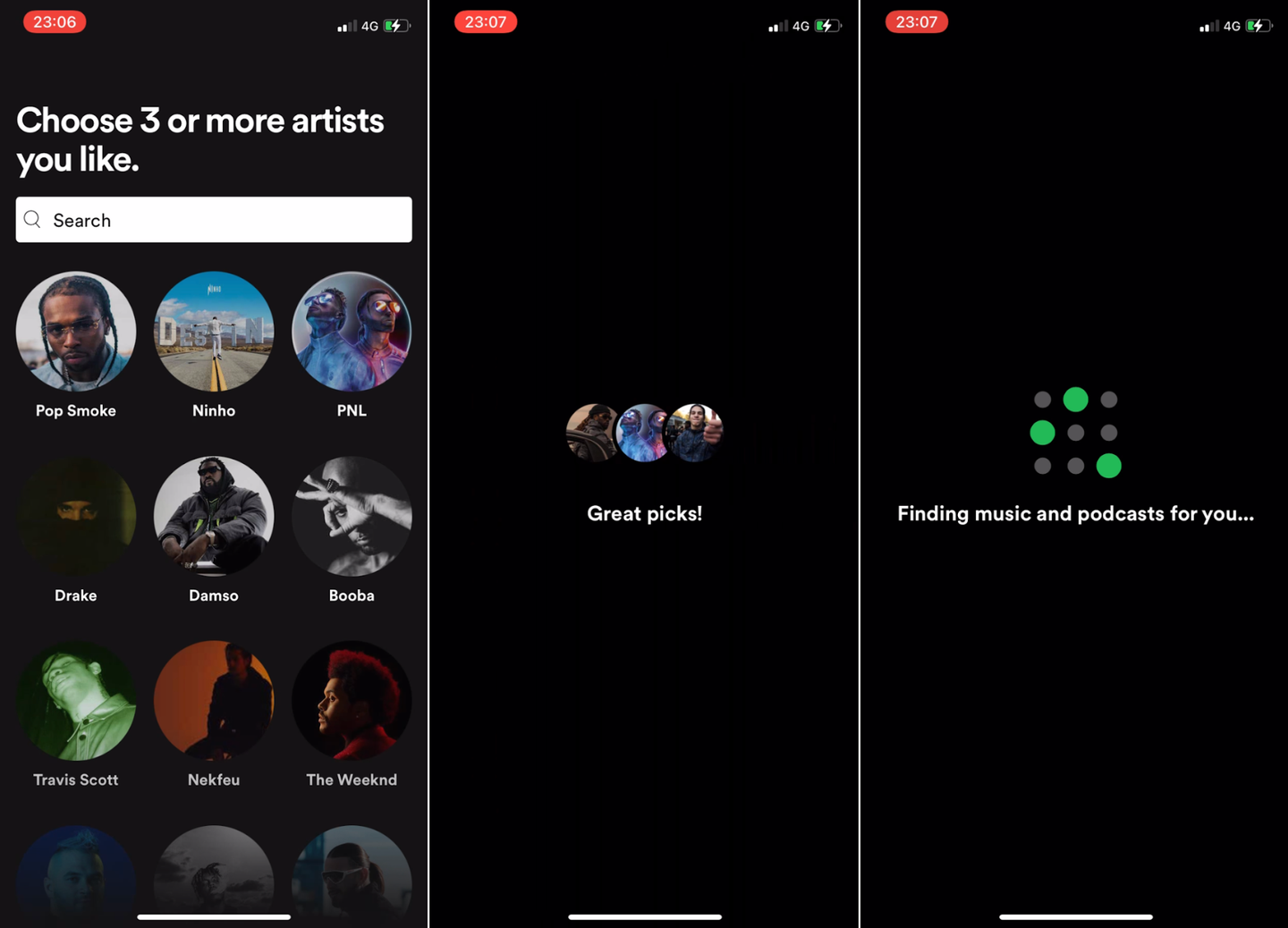
2. Intermediate: passive personalisation based on segments
This strategy removes the friction of active personalisation, by inferring customer preferences from usage data.
Passive personalisation takes a different approach, requiring no customer input. Instead, a customer’s experience is tailored based on inferences made from actual behavioural and interaction data. Where active personalisation can be achieved relatively straightforwardly (e.g. by tagging content, and displaying it based on stored user preferences), passive personalisation typically requires more sophisticated modelling.
South China Morning Post (SCMP) considered a range of models when creating their personalised content recommendations feature. Romain Rouquier, SCMP’s VP of Data, has explained the differences between the main approaches they considered:
- Content based filtering (assumption: if a user reads a specific story, they are likely to enjoy similar stories)
- Demographic based filtering (assumption: users sharing similar demographics are likely to enjoy similar stories)
- Collaborative filtering (assumption: readers sharing the same reading behaviour are likely to enjoy similar content)
Each of these models has strengths and weaknesses. For example, with content based filtering, you can’t be sure that because someone engaged with a piece of content that they enjoyed it. The second model relies on data that may be hard to access (e.g. if someone uses a VPN their location cannot be accurately inferred). Finally, while collaborative filtering has the strongest performance of these three methods, it requires extensive browsing history data across a userbase (sites with “fly by” visitors will struggle). As with most successful implementations of passive personalisation, SCMP has adopted a hybrid approach that builds on the strengths of all three models.
This modelling allowed SCMP to identify more than 20 user segments. Rouquier explains that these segments relate to key user personas, which include:
- Hong Kong readers who want local news.
- China watchers with an interest in in-depth analysis on policy and economics.
- Lifestyle enthusiasts exploring fashion and wellness content.
Each segment experiences SCMP in radically different ways – from the content they see, to their path to conversion. With ‘Lifestyle enthusiasts’, the publication focusses is on increasing serendipity with recommendations. ‘Hong Kong readers’ have a more immediate need for news, and are encouraged to subscribe far earlier on in their relationship with SCMP.
While there’s a greater complexity to this approach compared with Passive Personalisation, Rouqier believes that loyalty and engagement increase as the product and UX becomes more personalised: ‘These tools work, and they empower product and marketing while enhancing user experience.’
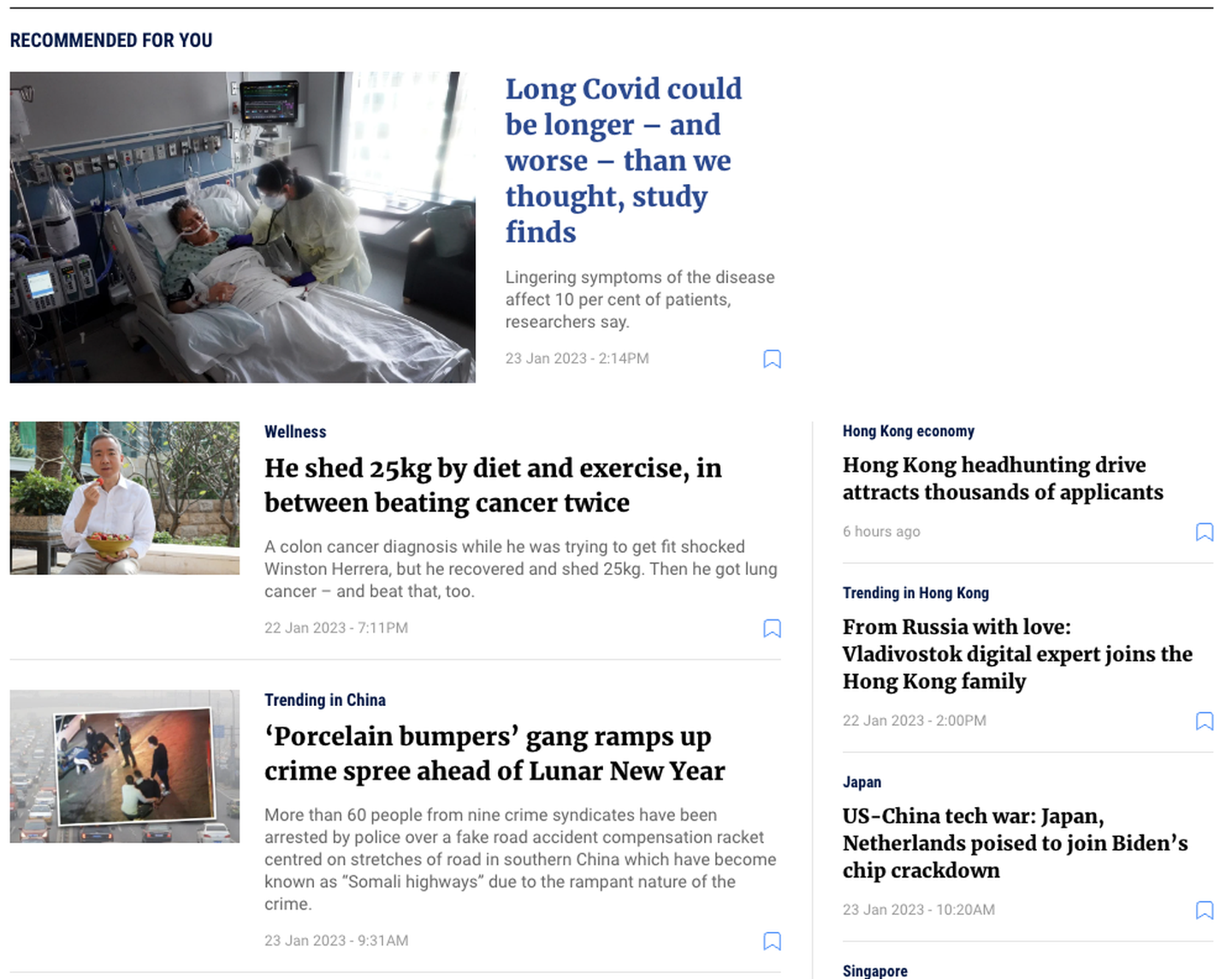
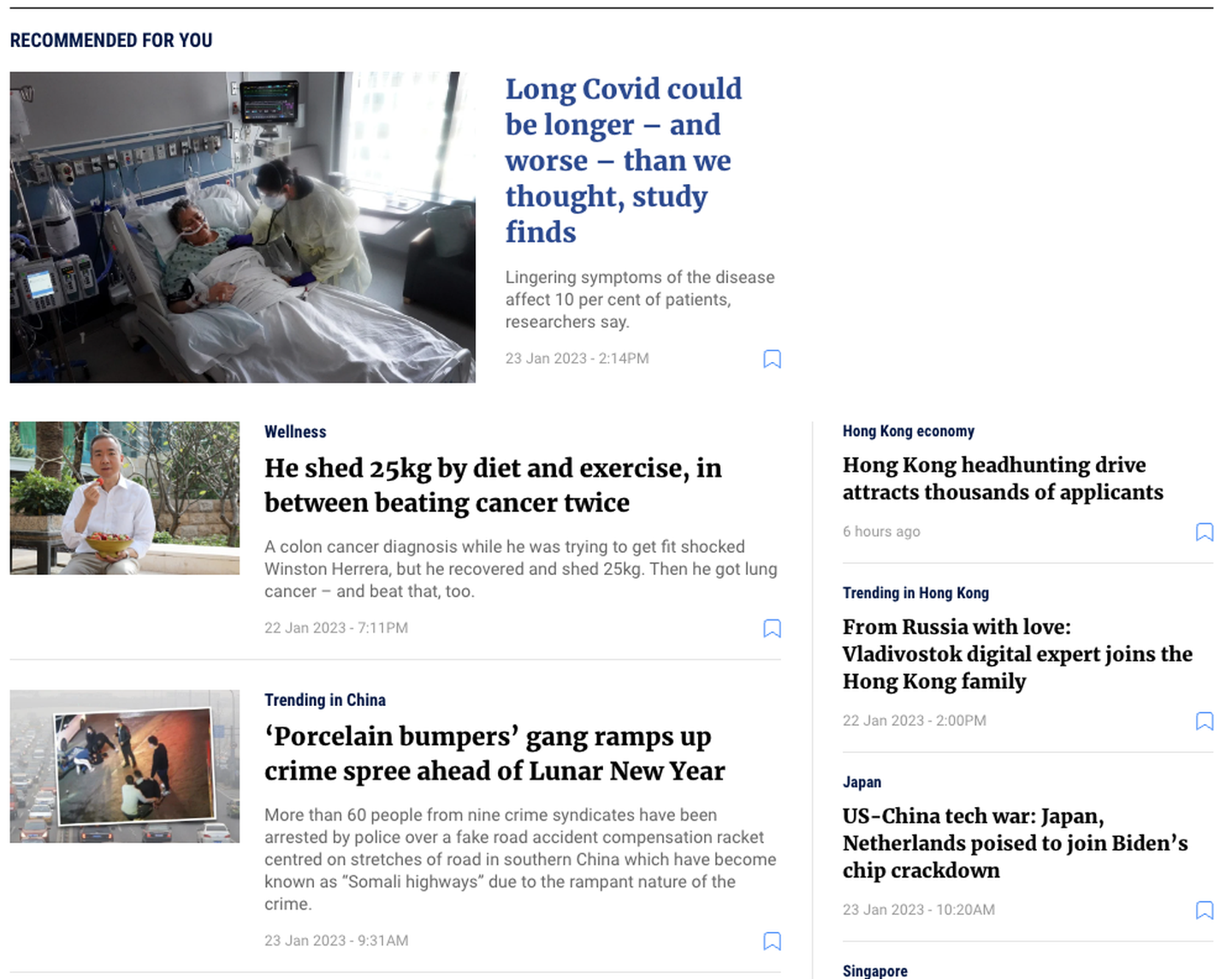
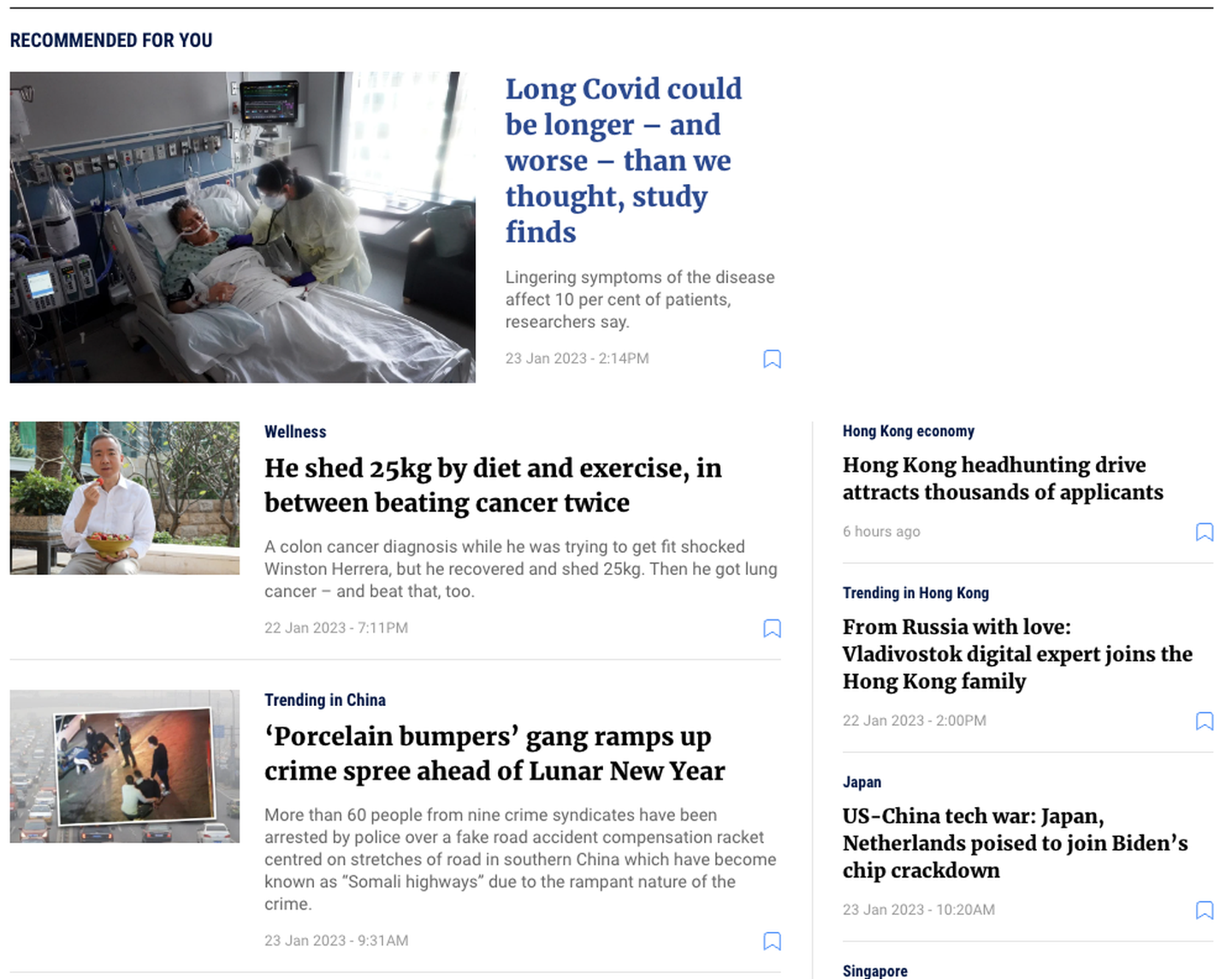
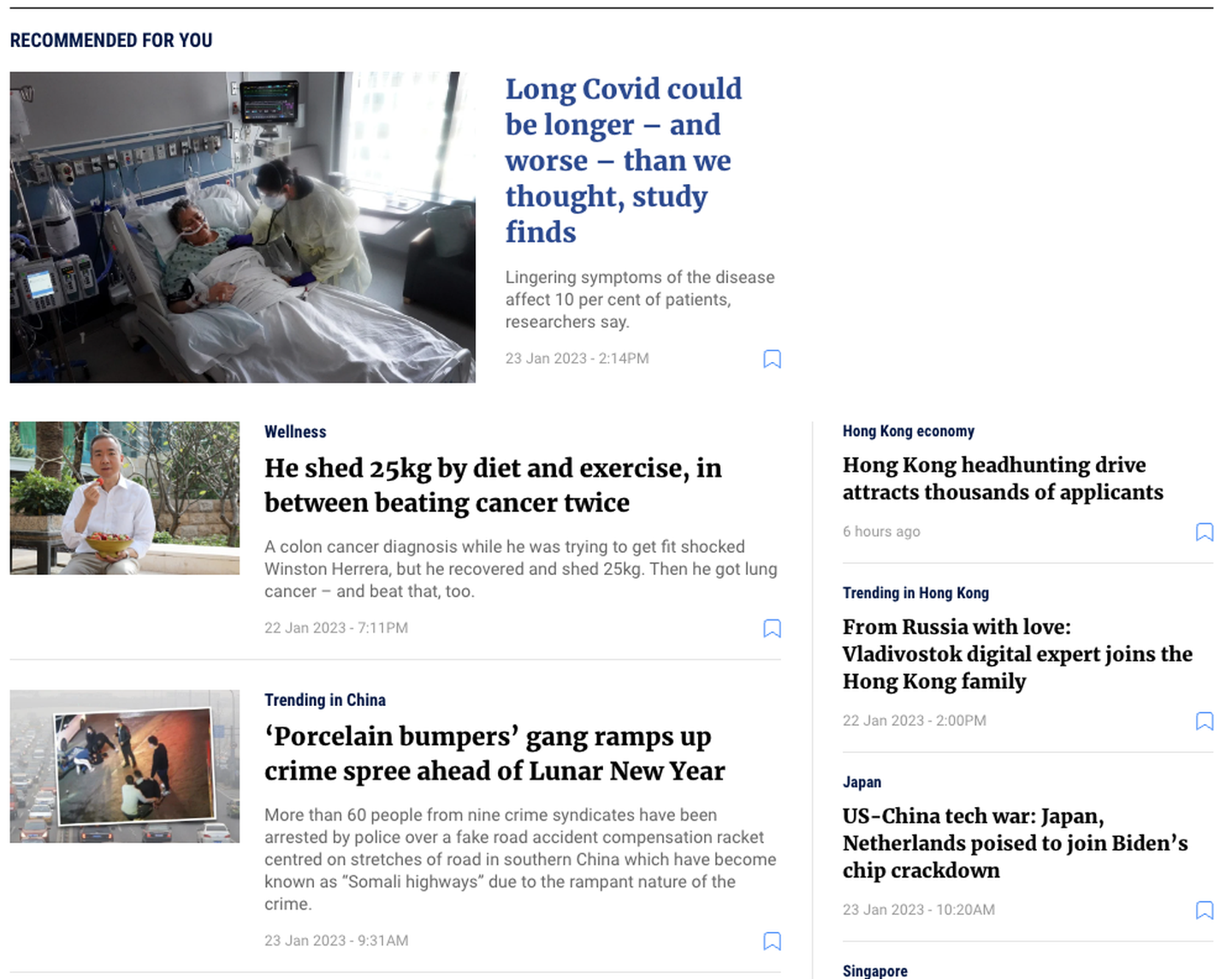
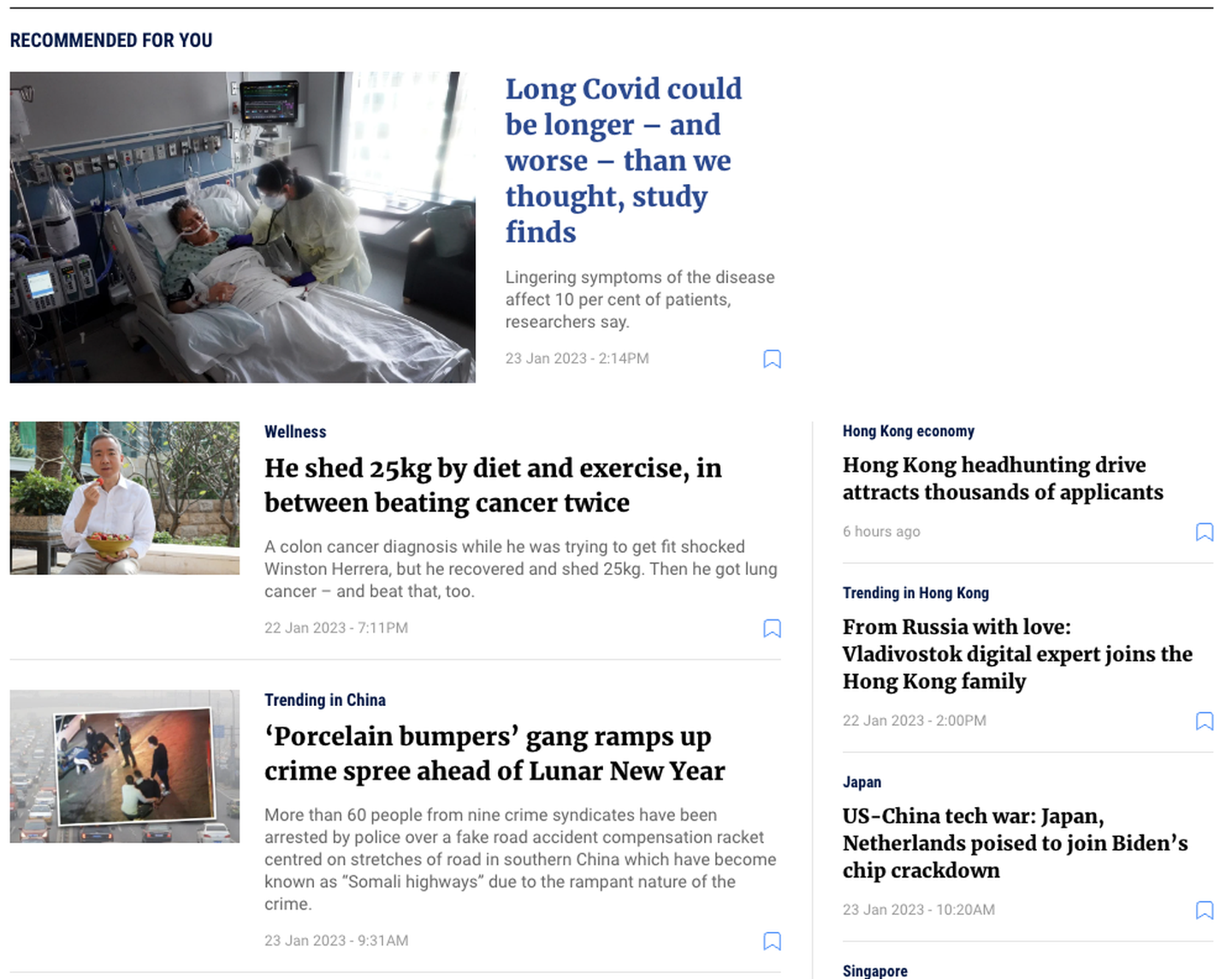
3. Advanced: passive personalisation that is truly individualised (‘Hyper-personalisation’)
The most technically sophisticated personalisation approach creates truly individualised experiences.
In more recent years, we’ve seen the rise of a sophisticated approach that some have labelled ‘hyper-personalisation’. For a taste what might be possible with personalisation, look no further than TikTok. The social video platform was 2022’s most-downloaded app worldwide. And at the heart of the TikTok experience is the ‘For You’ feed. This recommendation service is famed for becoming rapidly fine-tuned based solely on your interactions with the service.
Like the approach outlined by SCMP above, hyperpersonalisation is typically built based on hybrid datasets (e.g. content, demographics, collaborative filtering). But it goes far deeper in differentiating between individuals, treating each as a ‘segment-of-one’. To do this, it leverages sophisticated machine learning techniques rather than the more simple rules-based approach. This has the potential to create a truly unique experience for each user across the entire customer journey, from marketing and pricing, to customer service and loyalty.
Tech companies like Netflix, Spotify and Amazon are regarded as the early pioneers of hyperpersonalisation. Each of these services leans heavily on personalisation as a core part of their customer experience. For example, the Amazon homepage is unique to every user of the service. As well as bespoke widgets like ‘Keep shopping for’, ‘Buy again’ and ‘Pick up where you left off’, each user receives specific promotional banners based on what services and products you have previously used, bought or clicked on. Even the main menu adapts to you as an individual.
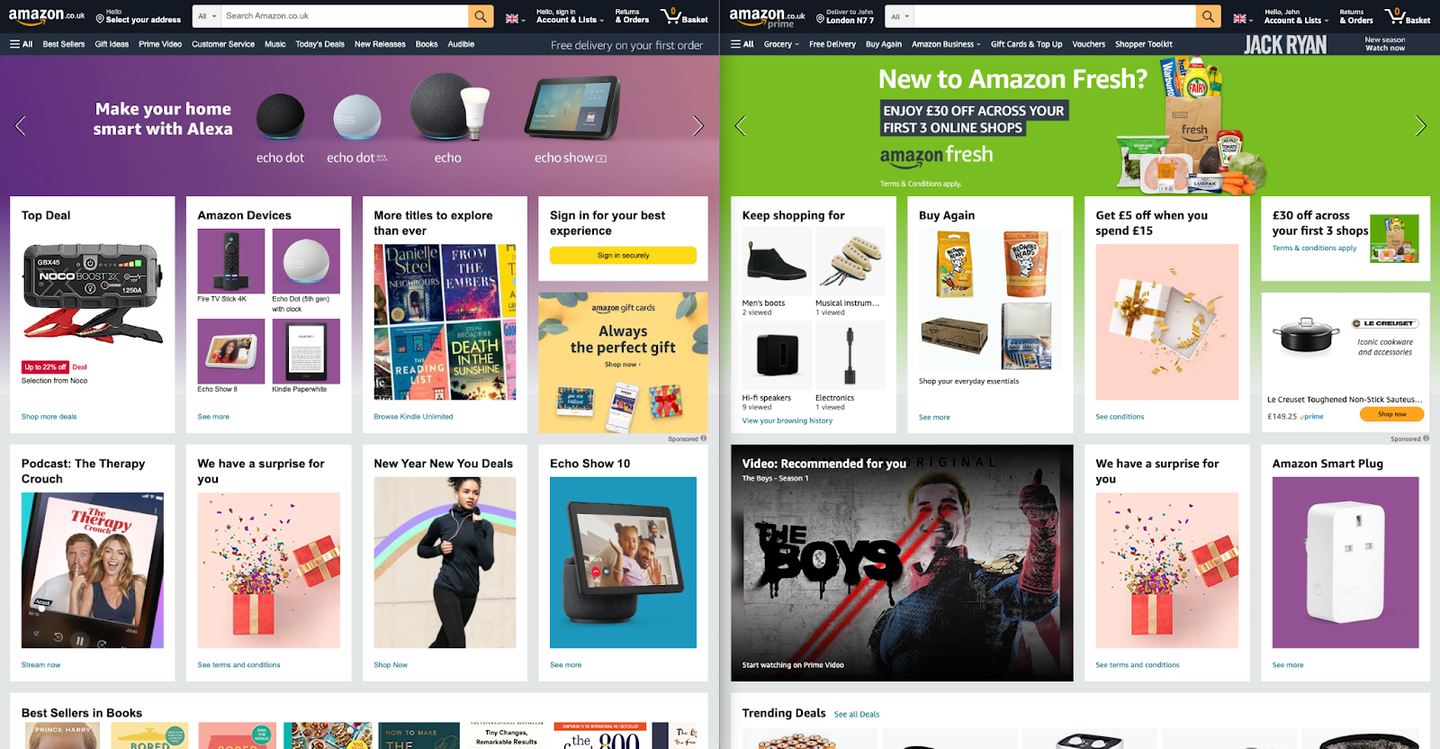
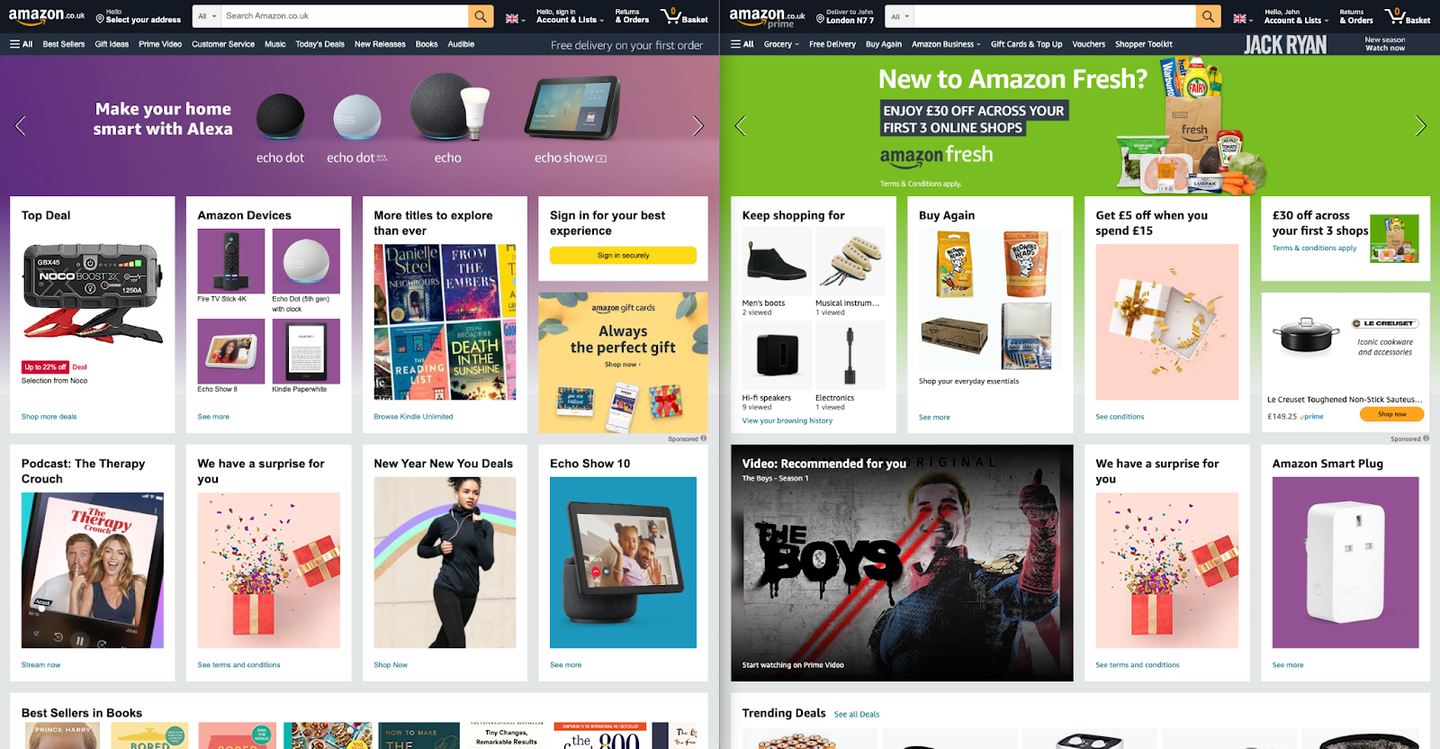
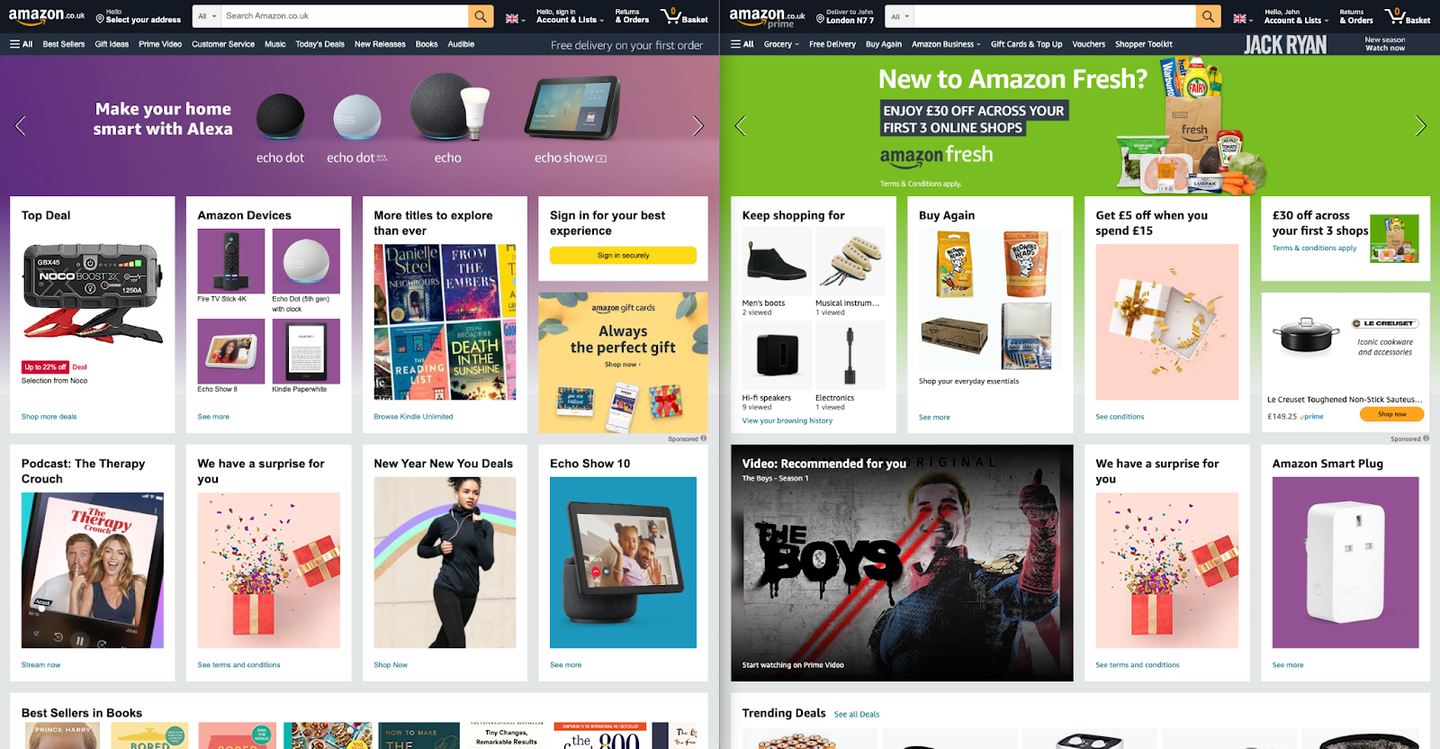
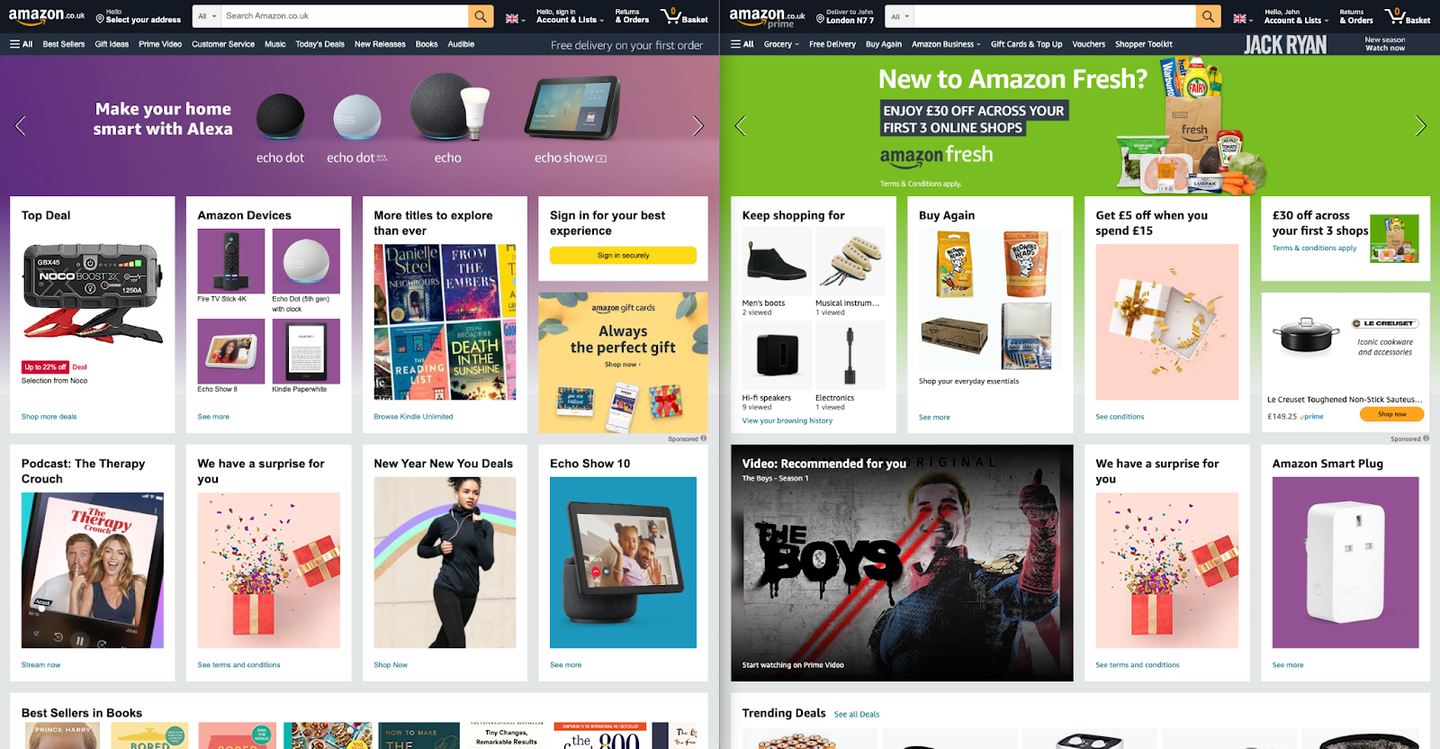
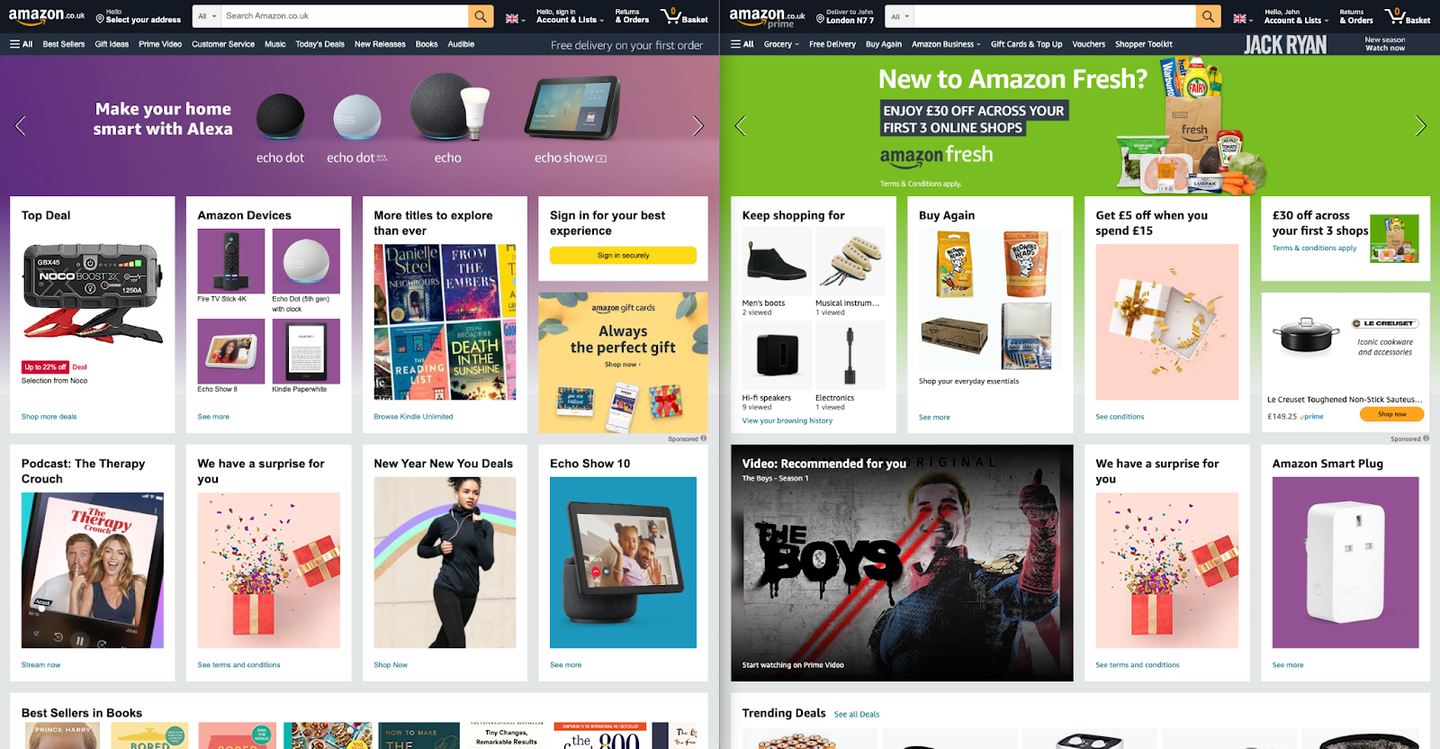
How personalisation can go wrong, and mitigating the risks.
All of the types of personalisation we’ve covered here involve customer data, and as such are subject to a rapidly evolving regulatory context. Personalisation also raises a specific concern around discrimination and bias (it isn’t hard to imagine what might go wrong with personalised pricing, for example). The risks are amplified when using passive techniques, where the mechanics and potential outputs of personalisation are less visible to users (and to some extent, your business). Fears of around personalisation and ‘surveillance’ have entered the mainstream, via feature films such as The Social Dilemmma.
To return to the stats that started this article, a huge majority of consumers expect and enjoy personalised interactions – despite an increasing awareness of what can go wrong. For this reason, investing in personalisation means making a commensurate investment in privacy and responsibility. There is no question that these tactics can backfire. However, a research study reported found Harvard Business Review found that these risks can be mitigated by three factors:
- Building trust by being transparent about the use of personal data in personalisation
- Giving users meaningful control over data sharing and personalisation
- Giving adequate justification about the purpose and benefits of data collection
For personalisation to be truly successful in the long term, we believe that these factors are just as important as your technical sophistication.
FT Strategies can help you implement a more mature approach to personalisation
We’ve helped clients from a range of sectors adopt personalisation in order to drive customer engagement, conversion and loyalty. We invite you to get in touch if you’d like to continue the conversation.