Interest in customer engagement has been growing ever since 2010 as demonstrated by increased search volumes on Google Trends, why?
Customer centric organisations, in every industry, are those that design their services and products with the end users in mind, striving to keep them happy and engaged.
An engaged customer is possibly more likely to return, share data and financially contribute to an organisation’s purpose through donations or subscriptions, for example, and do that for longer.
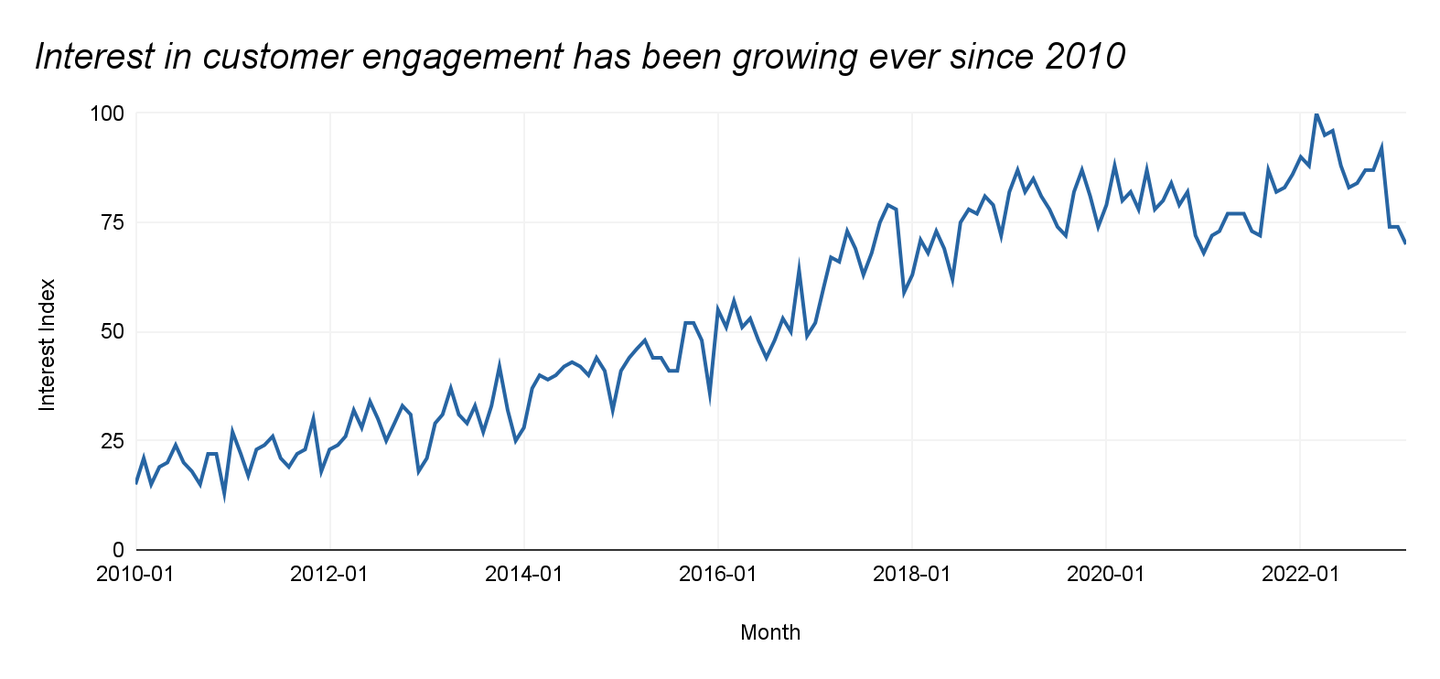
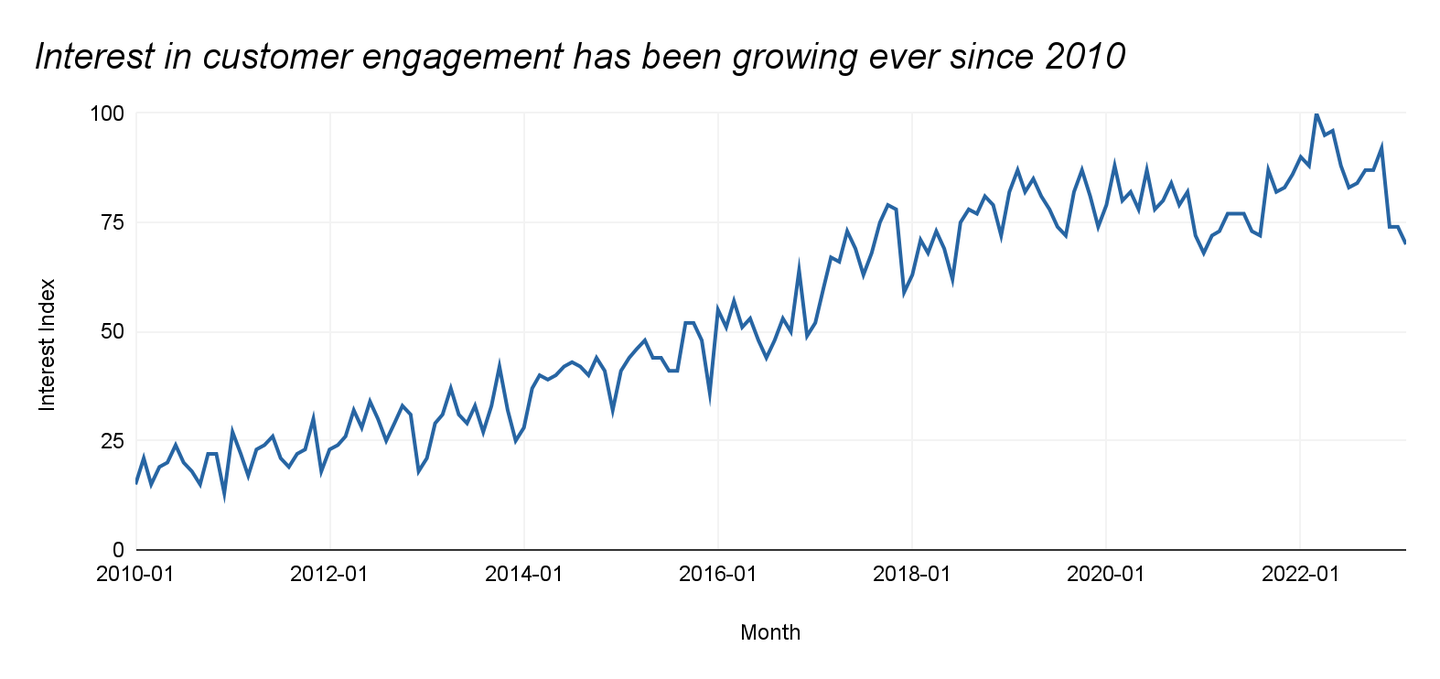
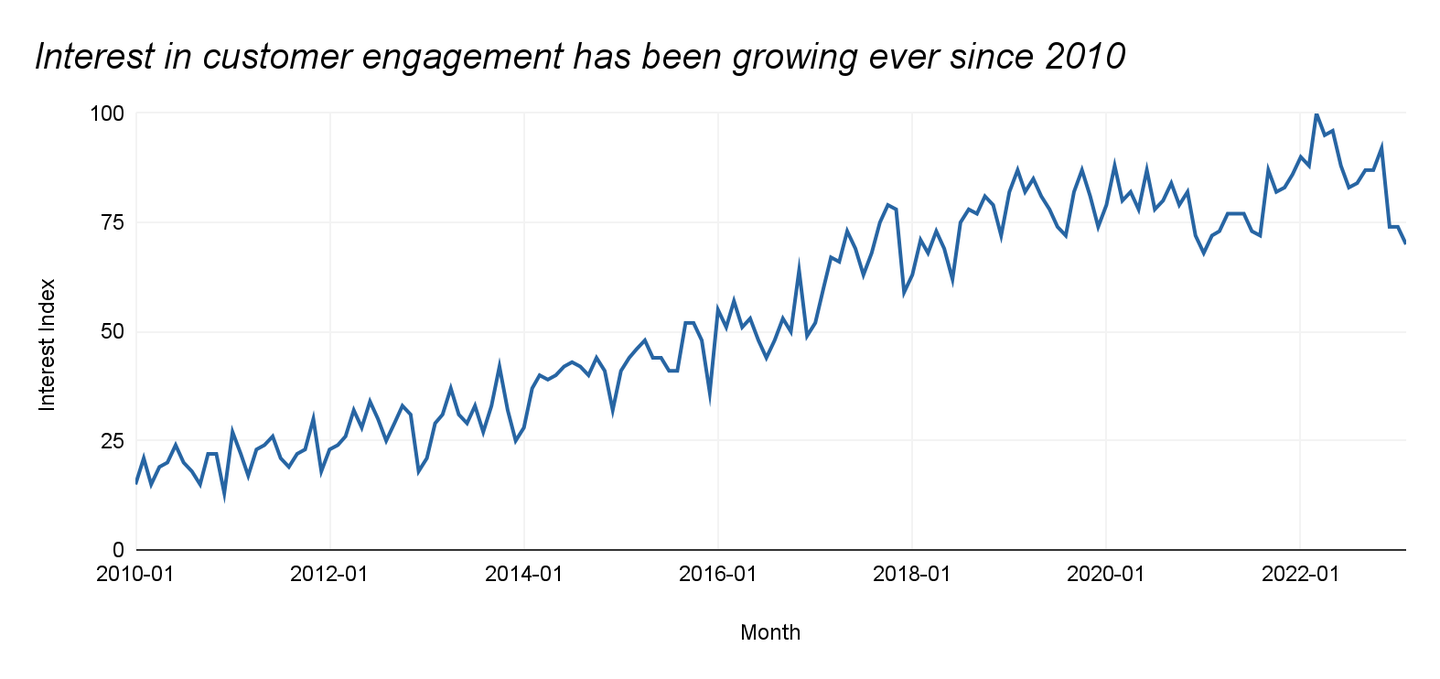
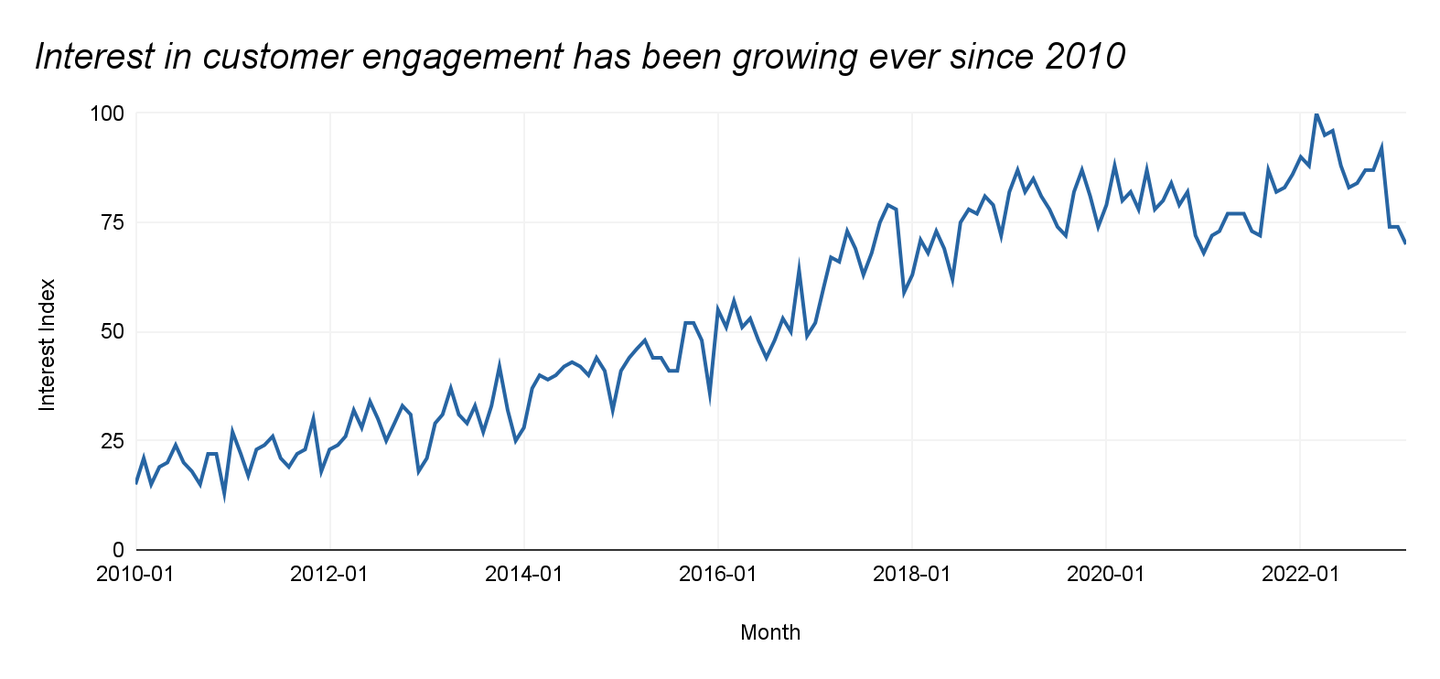
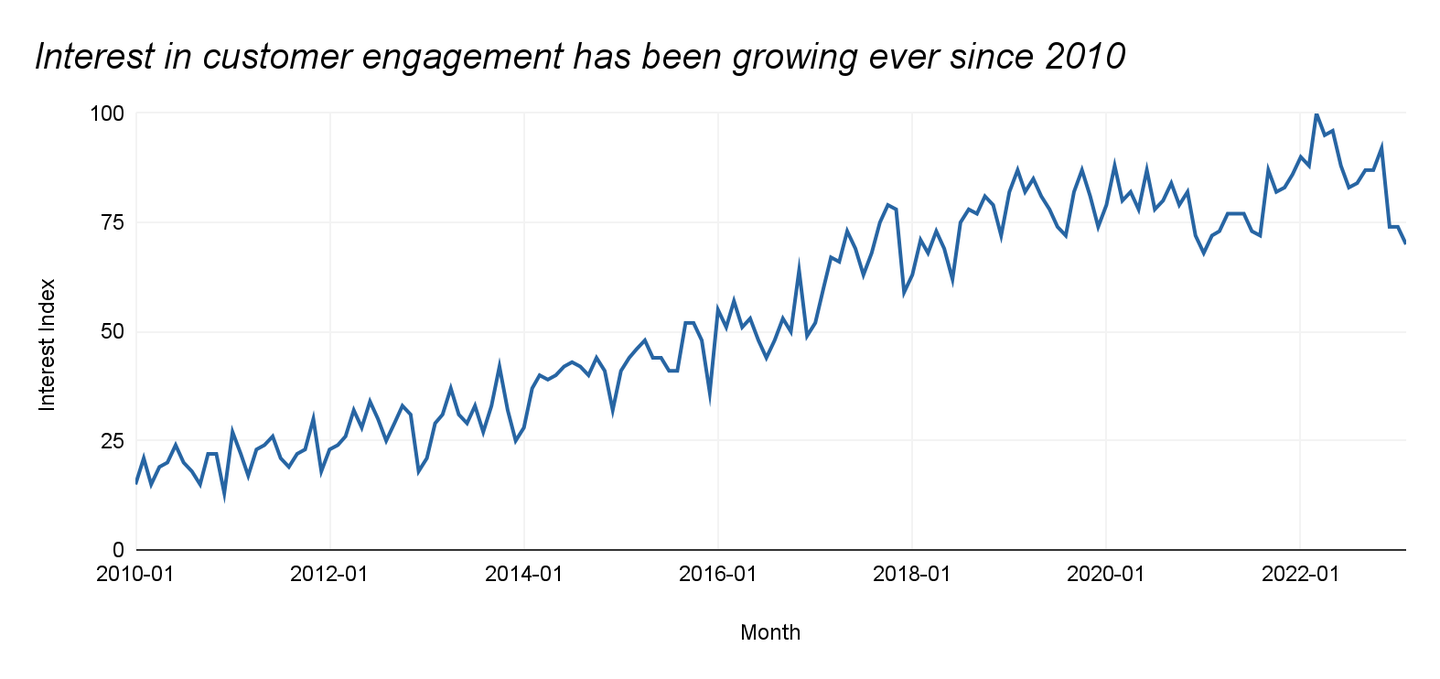
Measuring customer engagement and adopting a North Star metric to track progress can help by:
- Focusing on a single number when monitoring the ‘health’ of your audience
- Targeting acquisition, retention, up-selling and cross-selling efforts
- Measuring the commercial impact of new products and initiatives
From a data perspective, there are five pillars to building an engagement score (though not necessarily in this sequential order):
- Infrastructure: setting up the required data tracking and storing infrastructure
- Technical skills: building the appropriate data skills
- Framework: defining engagement and calculating the score
- Validation: validating the score against desired behaviours
- Implementation: productionising and leveraging the score
Let’s work through each of these pillars, one by one and showcase it with a real case study we at FT Strategies developed with one of our clients.
1. Infrastructure
The starting point is generally a data warehouse where all of the readers’ behavioural, profile and subscriptions data is stored, regularly updated and linked back to the individual readers records (note: this is more accurately achieved for known users navigating behind a login than for anonymous users). The idea is to be able to build a comprehensive view of users’ interactions with the products or services.
The level of granularity and latency of this data will define how sophisticated the metric can be. Below are some questions that may need to be considered.
- Does it include off-site interactions such as reading emails or playing videos and podcasts?
- Does it include the amount of time spent on a specific page?
- Is it updated every day?
2. Technical skills
Once the data is in the right place and format, any programming language such as SQL, R or Python, depending on what is available, can be used to develop the score.
A mix of Data Analysts and Data Scientists developed the RFV (our engagement metric) at the FT, originally in SQL (more on this here).
Depending on the structure and quality of the data, there may be additional cleaning, manipulation and aggregation required to build the baseline dataset. This dataset should provide an accurate and retrospective view of customers’ interactions on a daily basis (e.g. count of sessions and pageviews for each customer on each day in the past few months).
3. Framework
Starting with the assumption that “what is badly defined is likely to be badly measured”, it is worth spending time and effort coming up with a definition of what engagement means for our customers or readers.
This is a creative exercise with no perfect solution where inputs from the commercial, products and marketing teams are required.
Based on our experience at the FT as well as with some of our clients, customer engagement in the news publishing industry is usually defined based on content consumption. What we assume is that the more frequently users read, the higher the value they get out of our products, and as a result are more likely to stay longer and spend more.
“what is badly defined is likely to be badly measured”
Once the definition is clear, we need to calculate engagement. This is where the data team will look at the data points that are available and will identify which data points more accurately reflect the definition. If engagement is defined by the frequency and volume of consumption, then these are the two indicators that we need to extract from the data.
We advise organisations to start with a simple to build and easy to interpret metric and refine over time as and when required.
An example of a simple metric would be the ‘number of days in which a user was active over the last 30 days’. Whilst simple and straightforward, this metric generally carries enough information to provide the team with a good understanding of readers’ engagement (defined as consistent reading behaviour) and should hopefully correlate with retention, as shown in the next step.
4. Validation
Depending on the definition of engagement and the relevant data points that have been selected, there might be different ways to come up with a single engagement score. How do we know which one is right?
We create multiple versions of the score and we check their relationship against desired behaviours, for example by looking at the correlation between the engagement score and the retention rate. Then we select the one with the strongest positive relationship.
Going back to the simple metric mentioned earlier, we can create multiple versions of the score looking at different time frames, such as the last 30 days, last 60 days and last 90 days. From this we calculate the correlation between each of the scores and the retention rate and do this retrospectively.
Shown below is an example of a real case study we at FT Strategies developed with one of our clients. It demonstrates what the relationship between the engagement score (calculated over the last 30 days’ activity) and the churn rate could look like, if we group the engagement scores into 6 meaningful and mutually exclusive bands, which are as follows:
- Did not visit: 0 days
- Read less than once a week: < 5 days
- Read once or twice a week: 5 - 10 days
- Read every other day: 11 - 17 days
- Read almost every day: 18 - 25 days
- Read every day: 26 - 30 days
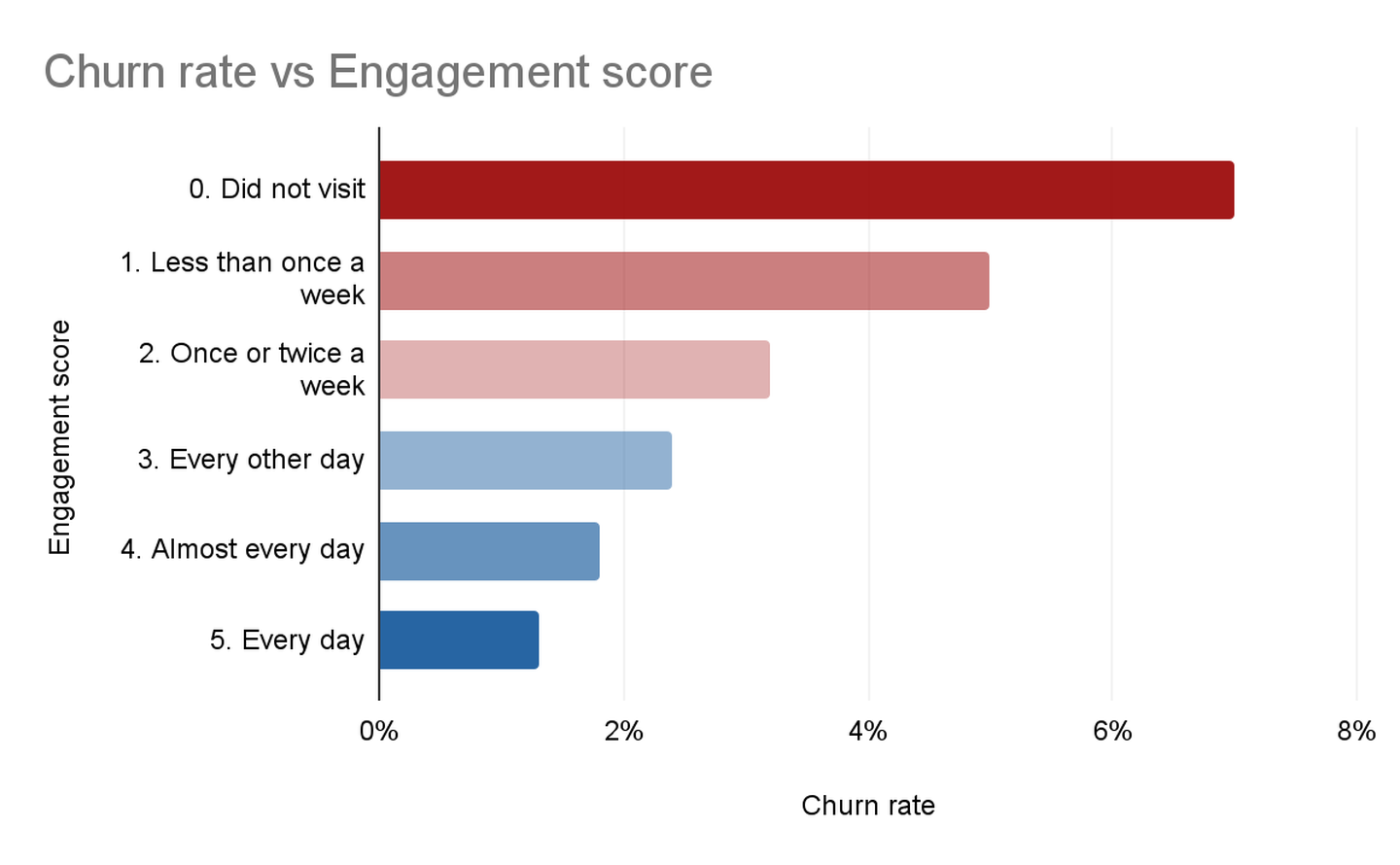
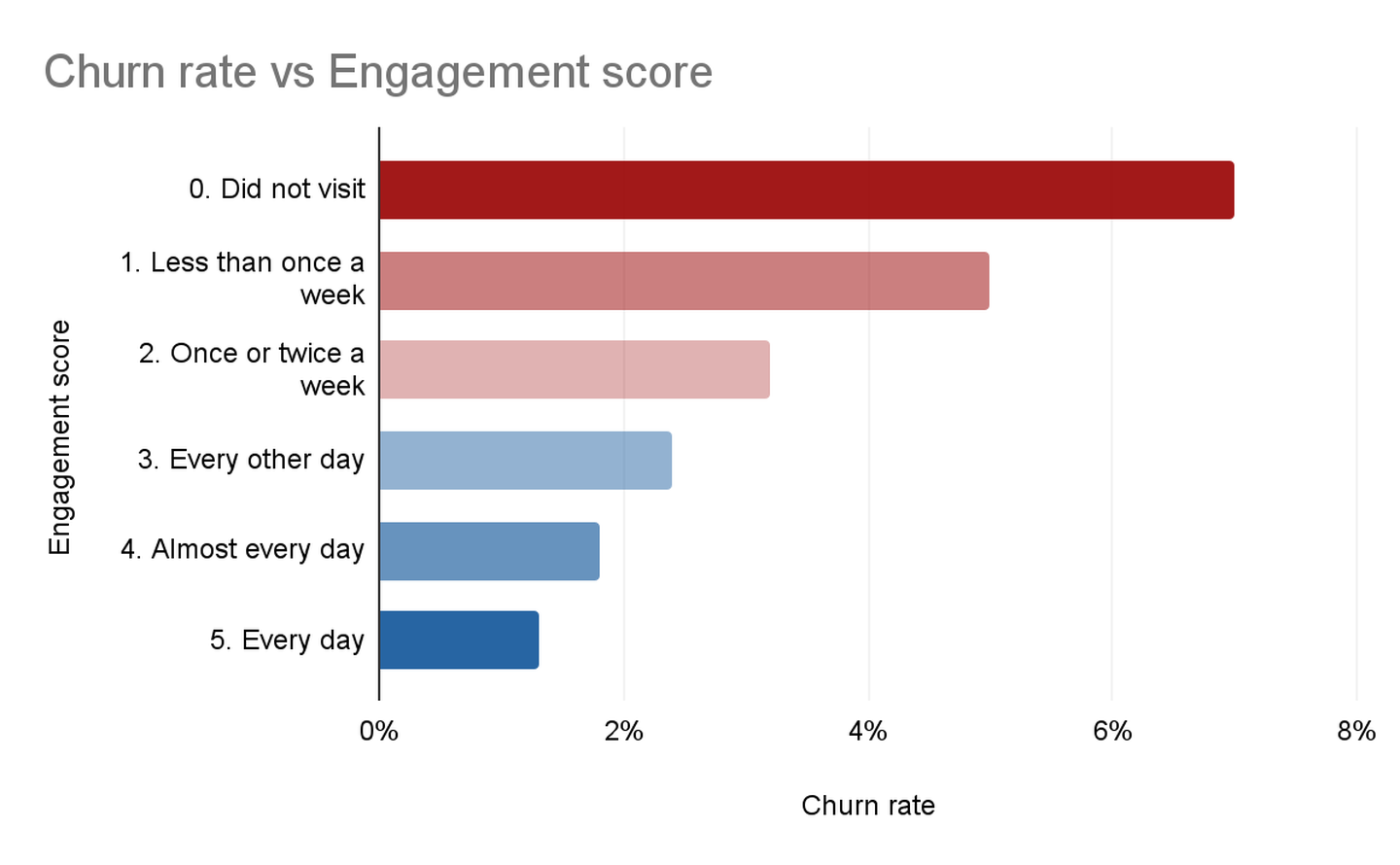
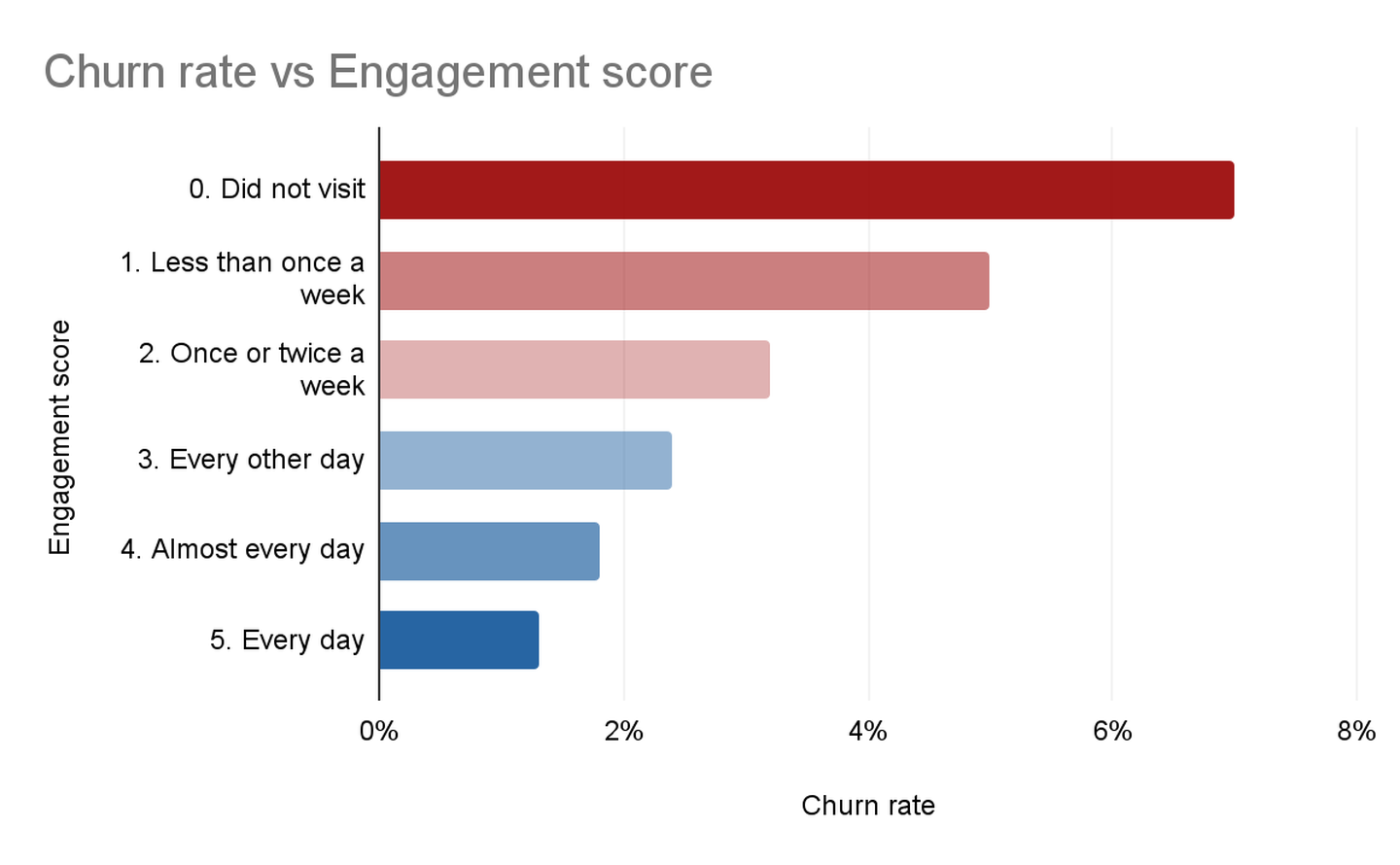
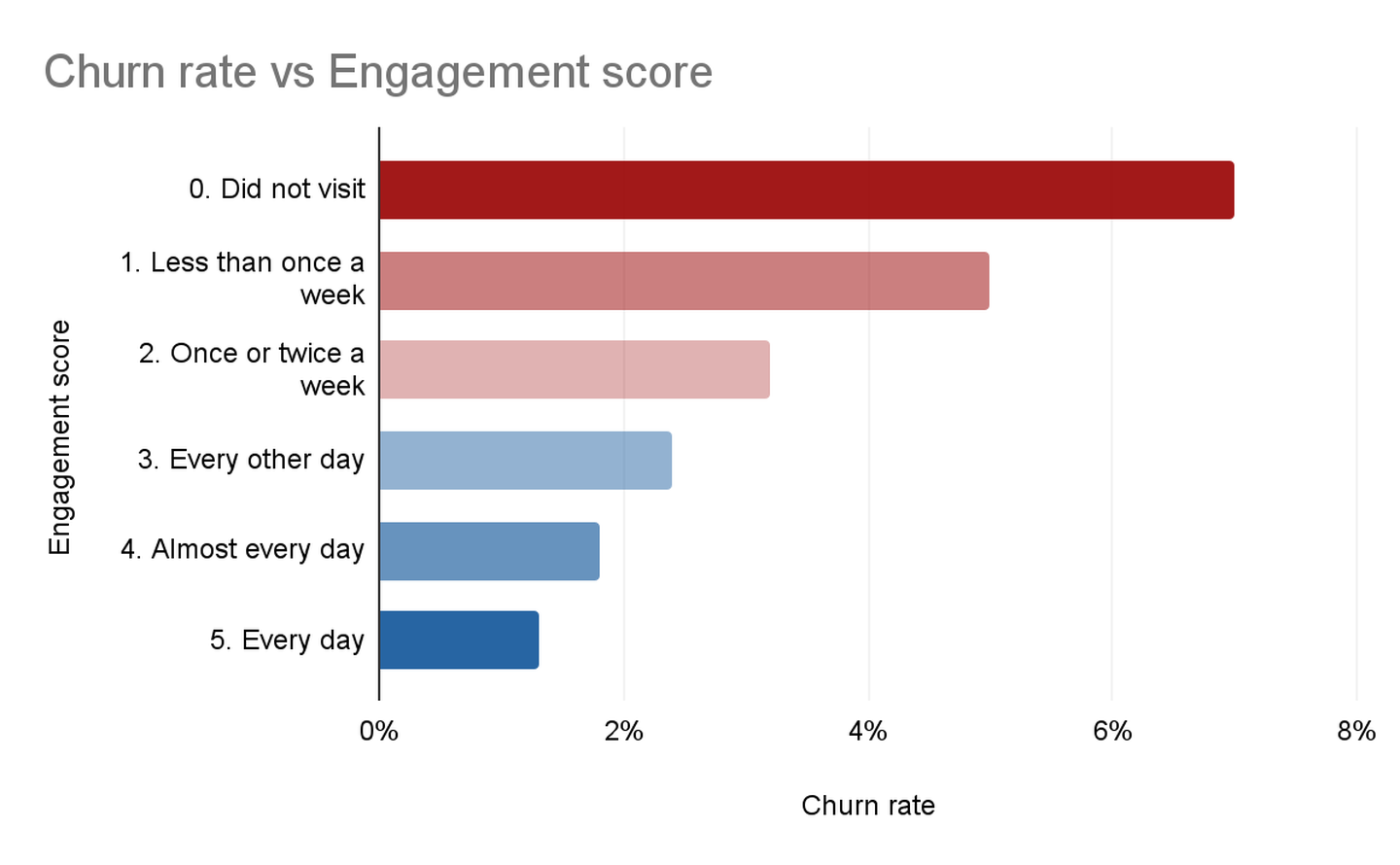
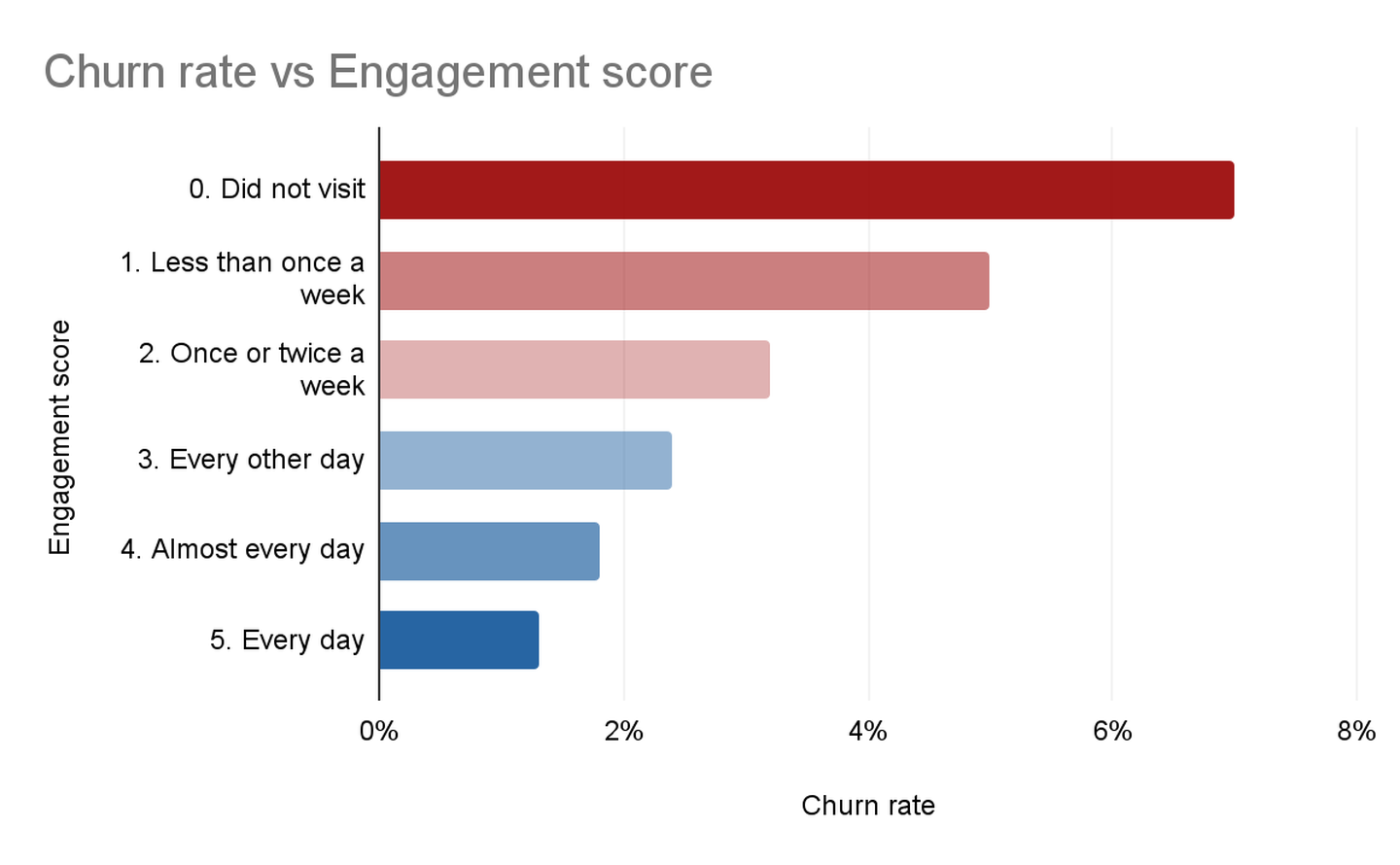
In this example, the relationship is very clear (i.e. the lower the engagement score the higher the chances of churn) and powerful enough to give us confidence in the validity of the engagement metric.
5. Implementation
How can you use the score? Here are a few ideas:
- Track the volume of engaged users over time (in the simple metric example, that could be those reading ‘Every other day’). Our original North Star metric at the FT was based on this metric.
- Create deep-dive analysis to understand what differentiates highly engaged users and disengaged ones? Do they use different or more products? Are they from different demographics groups? Does engagement form gradually over time and how long should it take for a user to become engaged?
- Implement personalised interventions to promote engagement and ultimately prevent churn. For example by building in-life campaigns to boost the engagement for those customers whose score is slowly dropping, by promoting exclusive features and/or highlighting the benefits of the products and services
Wrap Up
There are various ways of developing a customer engagement score, all valid but not necessarily right for your specific context. Every organisation should try and build a robust and transparent framework before embarking on this journey in order to maximise the chances of a successful implementation.
If you’d like to find out more of discuss anything mentioned in this blog, feel free to reach out to me directly.
How FT Strategies can help you
FT Strategies is the consultancy from the Financial Times that works with organisations to accelerate their digital transformation and power customer growth. The FT moved fast and invested proactively to build a best-in-class technology stack that underpins its digital-first business.
We are now sharing this expertise with organisations, in publishing, media and wider sectors, to help support their transition to digital-first businesses. If you want to find out more, please do not hesitate to reach out to us.
About the author
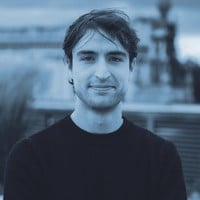