What type of content is being read by what type of visitor to your site? A deep knowledge of content consumption habits should be informing your content strategy. AI can help with this, says Aliya Itzkowitz, Manager at FT Strategies.
What have you learnt about using AI in content strategy?
AI is opening up new possibilities for content strategy as it enables a much richer picture of what content is resonating with our audience. Ways in which AI is helping include:
- Categorisation & tagging: automated data-labelling more accurately measures what types of articles you are producing (eg. by topic, storytelling format and user need).
- Real-time feedback: we can now understand what content is performing better in real-time. Whereas, in the past, there may have been a lag, AI-enhanced labelling and business intelligence tools make it possible to evaluate performance much faster.
- Personalisation: GenAI is now enabling rapid reformatting. This means content can be instantly repackaged and we can rapidly test which formats and ‘user needs’ serve each reader best.
In which use case has AI proved most effective?
We recently helped one European news publisher implement an AI tool to tag articles according to ‘user needs’. Their website had a lot of fly-by readers, so the goal of this project was to understand what content mix keeps readers engaged, so as to increase their loyalty.
To achieve this, their newsroom first wanted to understand content production and performance from a user needs standpoint. The goal of the user needs framework is to classify content based on what the reader gained from the article, eg. ‘update me’ or ‘educate me’. The newsroom wanted to know what user needs resonated most with readers so as to produce more of the content that was most engaging.
By using an off-the-shelf content analytics AI tool, the newsroom applied the user needs classification to a representative sample of 5,000 articles from the past six months. They decided to go with an external AI tool as they felt it would be a more efficient way to kick-start this project. The benefits of using AI here were that tagging was instant whilst manual tagging can take up to 10 minutes per article.
Initially, the project involved categorising content into relevant user needs groups. Next, the performance of each user need was analysed by connecting them to engagement metrics such as page views. Finally, the team conducted further research by segmenting the audience into cohorts (fly-by, loyal, heavy).
The AI-assisted analysis enabled the newsroom to understand content performance in a new way. The results showed that there was an opportunity to produce more emotive content. They appeared to be over-producing context-driven (‘understand’) and fact-driven (‘know’) articles. In fact, emotion-driven (‘feel’) and action-driven (‘do’) articles performed better — getting 40% and 20% more views on average but only accounting for 10% and 4% of all articles.
A secondary question of this experiment was: would the results be reliable? Would the AI-enabled tagging closely match tagging done by humans? We found that the human labellers often took a different view of what user needs category an article fell into. This is not to say that one set of labelling is more or less accurate than the other but highlights the need to adopt a clear classification and keep a human-in-the-loop to monitor results.
Now that the team has validated this approach, they are evaluating their options for how they can best operationalise user needs. For instance, they would like to create a live user needs dashboard for all journalists. They are also considering integrating the framework into the CMS so that user needs are considered holistically from the point of story ideation.
Three best practice top tips
- Ask Editorial for frequent feedback to tweak workflows. In the experiment, we found that the AI-enabled topic tagging did not always match the manual tagging by the newsroom. To reconcile differences, the editorial team provided detailed feedback to the tech vendor so as to adjust the settings of the model.
- Use your judgement to decide how to act on insights. As with any analysis, you can decide what next steps are appropriate. There are some kinds of stories that don’t perform well but that you may feel you have a moral duty to produce. One example in a lot of newsrooms we work with is coverage of the war in Ukraine. This topic has, generally, performed worse lately, but could be deemed essential coverage.
- Incorporate more nuanced engagement metrics. We started with page views, but they don’t tell the whole story. Particularly, if you’re trying to build deeper engagement, with a view to converting readers to subscribe, it’s important to track more metrics. If you can, try measuring aspects like scroll depth, dwell time or next action taken (eg. did the reader click on more stories next, or even subscribe).
If you would like to learn more about our AI solutions and how FT Strategies can help implement AI across your organisation, please get in touch here.
About the author
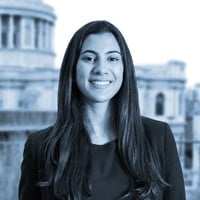
Aliya Itzkowitz is a Manager at FT Strategies, where she has worked with over 20 news companies worldwide. She previously worked at Dataminr, brining A.I. technology to newsrooms and at Bloomberg, as a journalist. She has a BA from Harvard University and an MBA from Said Business School, University of Oxford. She is currently a member of the FT's Next Generation Board.